Lane line detection method based on structural information
A lane line detection and structural information technology, applied in the field of computer vision and deep learning, can solve the problems of easy missing or false detection of lane lines, and achieve the effect of increasing the receptive field and strong generalization performance
- Summary
- Abstract
- Description
- Claims
- Application Information
AI Technical Summary
Problems solved by technology
Method used
Image
Examples
Embodiment Construction
[0064] The present invention will be further described below in conjunction with specific examples.
[0065] Such as figure 1 As shown, the lane line detection method based on structural information provided by the present invention is as follows:
[0066] Step 1. Obtain the lane line data set captured by the vehicle camera and divide it into training set, verification set and test set.
[0067] Step 2, converting the image and label data of the image dataset into the required format for the input of the deep convolutional neural network through preprocessing, including the following steps:
[0068] In step 2.1, the length of the input image and label is randomly scaled to the range of [256, 320], and the width is randomly scaled to the range of [768, 832]. For images of different input sizes, the scaled size should be adjusted accordingly.
[0069] In step 2.2, the scaled images and labels are randomly flipped horizontally with a probability of 0.5.
[0070] Step 2.3, rand...
PUM
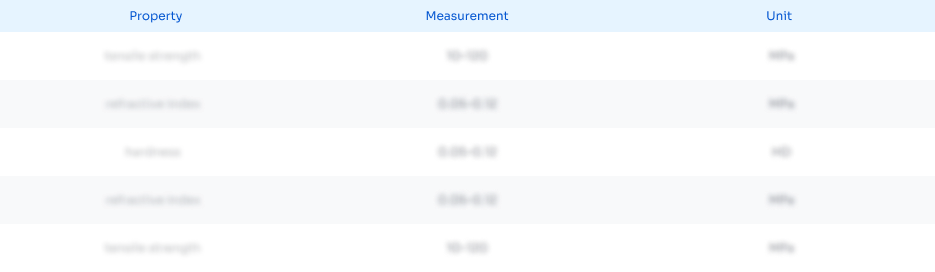
Abstract
Description
Claims
Application Information
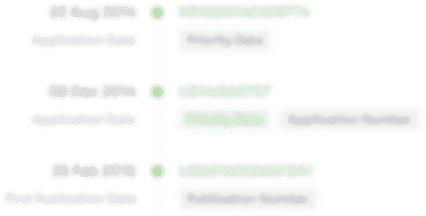
- R&D
- Intellectual Property
- Life Sciences
- Materials
- Tech Scout
- Unparalleled Data Quality
- Higher Quality Content
- 60% Fewer Hallucinations
Browse by: Latest US Patents, China's latest patents, Technical Efficacy Thesaurus, Application Domain, Technology Topic, Popular Technical Reports.
© 2025 PatSnap. All rights reserved.Legal|Privacy policy|Modern Slavery Act Transparency Statement|Sitemap|About US| Contact US: help@patsnap.com