A Hybrid System Energy Management Strategy Based on Inverse Deep Reinforcement Learning
A technology of reinforcement learning and energy management, applied in general control systems, control/regulation systems, instruments, etc., can solve problems such as inability to carry out online applications, and achieve fast calculation speed, good effect, and good real-time performance
- Summary
- Abstract
- Description
- Claims
- Application Information
AI Technical Summary
Problems solved by technology
Method used
Image
Examples
Embodiment
[0039] Such as figure 1 , figure 2 As shown, a hybrid system energy management strategy based on reverse deep reinforcement learning includes the following steps:
[0040] S1: Use the optimization solution method to calculate the global hybrid mode allocation ratio and the global optimized SOC result under one of the complete working conditions, and form an expert state-action pair as expert knowledge for reverse reinforcement learning; the optimization solution method includes Pseudospectral method, dynamic programming method, genetic algorithm.
[0041] S2: Create a reward function neural network and initialize parameters;
[0042] The reward function neural network is composed of a fully connected neural network, a convolutional neural network, and a long-term short-term memory neural network stacked in the order of a convolutional neural network, a long-term short-term memory neural network, and a fully connected neural network; the fully connected neural network consis...
PUM
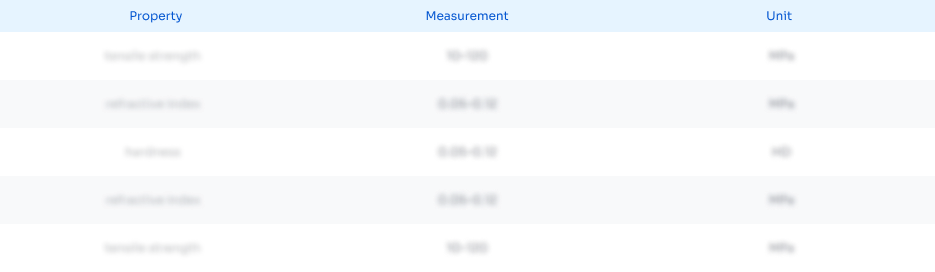
Abstract
Description
Claims
Application Information
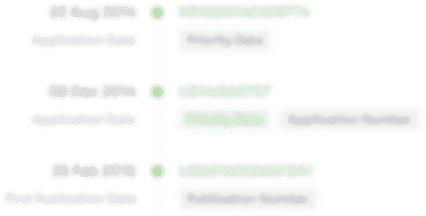
- R&D Engineer
- R&D Manager
- IP Professional
- Industry Leading Data Capabilities
- Powerful AI technology
- Patent DNA Extraction
Browse by: Latest US Patents, China's latest patents, Technical Efficacy Thesaurus, Application Domain, Technology Topic, Popular Technical Reports.
© 2024 PatSnap. All rights reserved.Legal|Privacy policy|Modern Slavery Act Transparency Statement|Sitemap|About US| Contact US: help@patsnap.com