Neural network model quantification method and device and electronic equipment
A neural network model and technology of electronic equipment, applied in the field of machine learning, can solve problems such as high computational complexity and inability to run target equipment
- Summary
- Abstract
- Description
- Claims
- Application Information
AI Technical Summary
Problems solved by technology
Method used
Image
Examples
Embodiment 1
[0035] First, refer to figure 1 An example electronic device 100 for implementing a neural network model quantization method, apparatus and electronic device according to an embodiment of the present invention will be described.
[0036] like figure 1 Shown is a schematic structural diagram of an electronic device. The electronic device 100 includes one or more processors 102, one or more storage devices 104, an input device 106, an output device 108, and an image acquisition device 110. These components pass through a bus system 112 and / or other forms of connection mechanisms (not shown). It should be noted that figure 1 The components and structure of the electronic device 100 shown are only exemplary, not limiting, and the electronic device may also have other components and structures as required.
[0037] The processor 102 can be implemented in at least one hardware form of a digital signal processor (DSP), a field programmable gate array (FPGA), and a programmable log...
Embodiment 2
[0044] This embodiment provides a method for quantifying a neural network model, which can be executed by the above-mentioned electronic equipment such as a computer, see figure 2 The flow chart of the method for quantifying the neural network model is shown, the method mainly includes the following steps S202 to S208:
[0045] Step S202, obtaining a neural network model; wherein, the neural network model includes a convolutional layer, a normalization layer, and a quantized activation layer, and the output features of the quantized activation layer are integer features.
[0046] The above-mentioned neural network model can be a model that completes the training of a quantized neural network model, and the neural network model can be a convolutional neural network, wherein the convolutional layer, normalization layer, and quantization activation layer in the convolutional neural network network layer are sequentially connection, since there are still floating-point parameters...
Embodiment 3
[0085] On the basis of the foregoing embodiments, this embodiment provides an example of applying the foregoing neural network model quantification method to quantify the convolutional neural network, see e.g. image 3 The flow chart of convolutional neural network quantization shown in the figure can be executed with reference to the following steps S302 to S306:
[0086] Step S302: During the iterative training of the convolutional neural network, the parameters of the target network layer of the convolutional neural network are quantized until the iterative training ends, and a trained convolutional neural network is obtained.
[0087] The above-mentioned target network layer includes a convolutional layer, a normalization layer, and a quantized activation layer, and may also include other network layers. During the training phase of a convolutional neural network, see e.g. Figure 4 The flow chart of parameter dumping and deployment of the convolutional neural network is ...
PUM
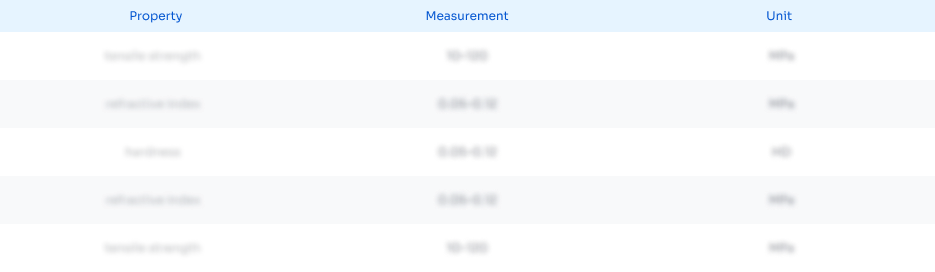
Abstract
Description
Claims
Application Information
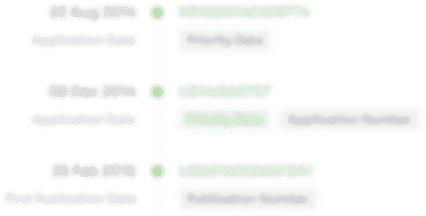
- R&D Engineer
- R&D Manager
- IP Professional
- Industry Leading Data Capabilities
- Powerful AI technology
- Patent DNA Extraction
Browse by: Latest US Patents, China's latest patents, Technical Efficacy Thesaurus, Application Domain, Technology Topic.
© 2024 PatSnap. All rights reserved.Legal|Privacy policy|Modern Slavery Act Transparency Statement|Sitemap