RNA binding protein recognition method based on multi-view depth features and multi-label learning
A deep-featured, protein-binding technology used in the field of RNA-binding protein recognition
- Summary
- Abstract
- Description
- Claims
- Application Information
AI Technical Summary
Problems solved by technology
Method used
Image
Examples
Embodiment 1
[0075] Following the implementation of the training phase, the examples were done for the RNA-RBP binding data of the AURA2 dataset. The dataset contains 67 RBPs and 73681 RNA sequences and their 550386 binding site information, as shown in Table 1. The amount of sample RNA that can be bound by each RBP is different and varies greatly. The length of each RNA sequence is different, so we uniformly stipulated a length of 2700, and the deficiency was filled with base B. Table 2 shows the comparison results of the method iDeepMV used in the present invention and the current advanced methods in this field.
[0076]
[0077]
[0078] The performance index of this algorithm in the embodiment 1 of table 2
[0079]
[0080] Among them, RNA perspective-, amino acid perspective-, dipeptide perspective- and voting results- are the prediction results of the neural network that have not been trained by the multi-label classifier in the iDeepMV method and their voting results, RNA...
Embodiment 2
[0083] In order to reflect the prediction accuracy of the method of the present invention from an individual, Table 3 calculates the prediction effect of the method used in this experiment and the advanced method in this field on different RBPs.
[0084] Table 3 Prediction effects of different RBPs
[0085]
[0086]
[0087]
[0088] The abscissa of the three graphs in Figure 13 is the number of samples of different RBPs, and the ordinate is the precision rate, recall rate and F1-score respectively. It can be seen that, with the gradual increase in the number of samples of the three methods, each index shows a gradual increase and a flattening trend. It is noticed that when the number of samples is less than 5000, the fluctuations of various indicators are very large. This is because the number of samples of some classes is too small and the model cannot learn the deep features of these samples well. And from the comparison of the three curves, the learning ability o...
PUM
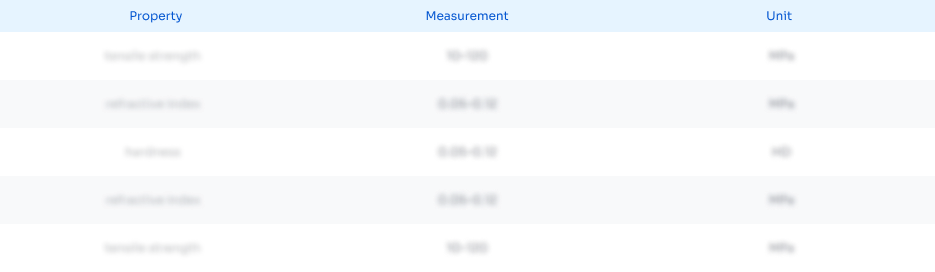
Abstract
Description
Claims
Application Information
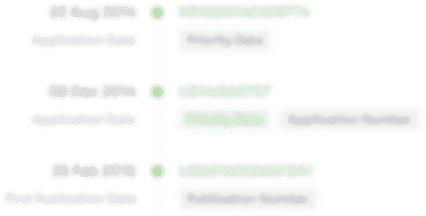
- R&D
- Intellectual Property
- Life Sciences
- Materials
- Tech Scout
- Unparalleled Data Quality
- Higher Quality Content
- 60% Fewer Hallucinations
Browse by: Latest US Patents, China's latest patents, Technical Efficacy Thesaurus, Application Domain, Technology Topic, Popular Technical Reports.
© 2025 PatSnap. All rights reserved.Legal|Privacy policy|Modern Slavery Act Transparency Statement|Sitemap|About US| Contact US: help@patsnap.com