Brain-control hybrid intelligent rehabilitation method based on novel deep learning model
A technology of deep learning and rehabilitation methods, applied in neural learning methods, biological neural network models, medical science, etc., can solve the problems of small amount of effective data, difficult to train models, and affect the accuracy of model training, etc., to achieve improved results Effect
- Summary
- Abstract
- Description
- Claims
- Application Information
AI Technical Summary
Problems solved by technology
Method used
Image
Examples
Embodiment Construction
[0025] A brain-controlled hybrid intelligent rehabilitation method based on a novel deep learning model of the present invention will be described in detail below in conjunction with the embodiments and drawings.
[0026] Such as figure 1 As shown, a brain-controlled hybrid intelligent rehabilitation method based on a new deep learning model of the present invention first obtains the subject's motor imagery EEG signals through a brain-computer interface composed of an electrode cap and a portable EEG signal acquisition device, The motion intention of the subject is decoded by the decoding model; the rehabilitation equipment is controlled according to the motion intention of the subject to assist the subject to perform limb movements; As the training progresses, the decoding model is constantly updated to adapt to changes in the EEG characteristics of the test subject; the training data set is derived from a motor imagery database, and the motor imagery database is used to stor...
PUM
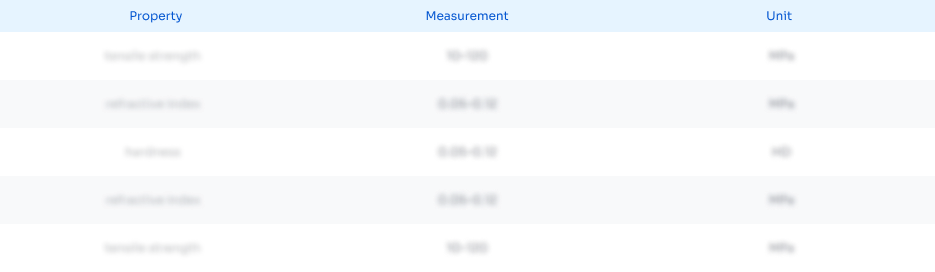
Abstract
Description
Claims
Application Information
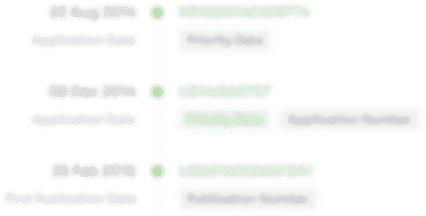
- R&D Engineer
- R&D Manager
- IP Professional
- Industry Leading Data Capabilities
- Powerful AI technology
- Patent DNA Extraction
Browse by: Latest US Patents, China's latest patents, Technical Efficacy Thesaurus, Application Domain, Technology Topic.
© 2024 PatSnap. All rights reserved.Legal|Privacy policy|Modern Slavery Act Transparency Statement|Sitemap