Multi-dimensional continuous optimization variable global optimization method based on reinforcement learning
A technology of optimizing variables and reinforcement learning, which is applied in the field of optimization algorithms, can solve problems such as slow convergence speed and inability to support large-scale optimization variables, and achieve the effect of reducing manual participation
- Summary
- Abstract
- Description
- Claims
- Application Information
AI Technical Summary
Problems solved by technology
Method used
Image
Examples
Embodiment
[0028] The entire optimization process is as follows figure 1 The overall flow of the global optimization algorithm shown is performed. First select the aircraft wing airfoil in aeronautics as the object, and optimize its aerodynamic shape. The optimization goal is to increase its lift coefficient while keeping the drag coefficient constant, and use the free-form deformation technology (FFD) method to parameterize it .
[0029] Then build a reinforcement learning environment based on Bayesian optimization algorithm and optimization effect evaluation algorithm. For Bayesian optimization algorithms, use Python to write calculation files, which include optimization variables, airfoil deformation methods, aerodynamic calculations, as well as Bayesian optimization algorithm calls and optimization results output; for optimization evaluation algorithms, use Python language According to the objective function and constraint conditions of the specific optimization problem, a suitable qu...
PUM
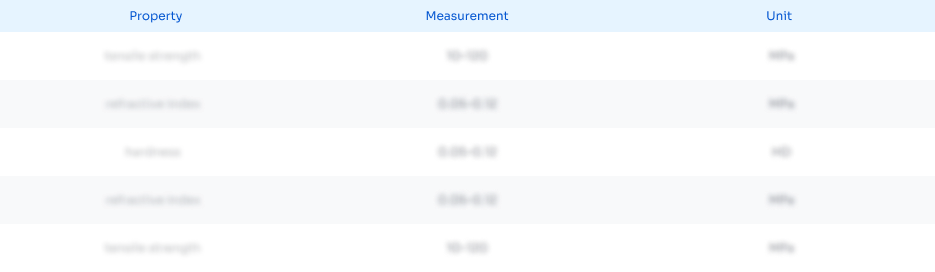
Abstract
Description
Claims
Application Information
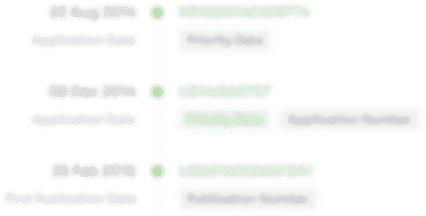
- R&D Engineer
- R&D Manager
- IP Professional
- Industry Leading Data Capabilities
- Powerful AI technology
- Patent DNA Extraction
Browse by: Latest US Patents, China's latest patents, Technical Efficacy Thesaurus, Application Domain, Technology Topic, Popular Technical Reports.
© 2024 PatSnap. All rights reserved.Legal|Privacy policy|Modern Slavery Act Transparency Statement|Sitemap|About US| Contact US: help@patsnap.com