Progressive ensemble classification method based on kernel width learning system
A technology of learning system and classification method, applied in the field of progressive integrated classification based on kernel width learning system, can solve the problem of cumbersome feature engineering work, and achieve the effect of reducing the cost of manual design features, improving the generalization ability, and improving the classification effect.
- Summary
- Abstract
- Description
- Claims
- Application Information
AI Technical Summary
Problems solved by technology
Method used
Image
Examples
Embodiment Construction
[0053] The present invention will be further described below in conjunction with specific examples.
[0054] Such as figure 1 As shown, the progressive integrated classification method based on the kernel width learning system provided in this embodiment includes the following steps:
[0055] 1) Input training samples and test samples. The samples contain desensitized gene features and corresponding labels (various traits), and preprocess the biological gene data in the samples. The specific preprocessing includes the following steps:
[0056] 1.1) Data cleaning of biological genetic data, including median filling of data with missing values and deletion of attributes with a large number of missing values, and unification of data types and data normalization;
[0057] 1.2) Convert the category label of the sample into a one-hot encoding to facilitate subsequent calculations. One-hot encoding is One-hot encoding, also known as one-bit effective encoding. The conversion proces...
PUM
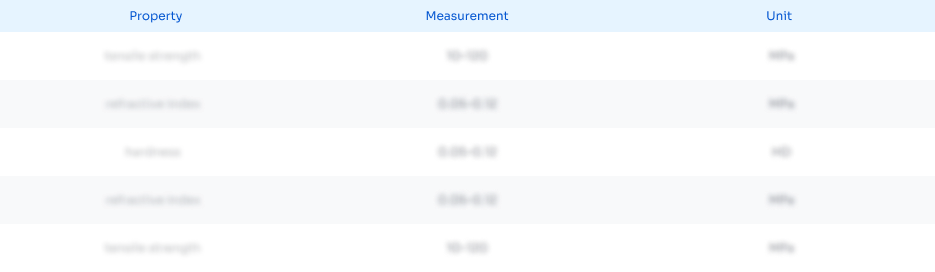
Abstract
Description
Claims
Application Information
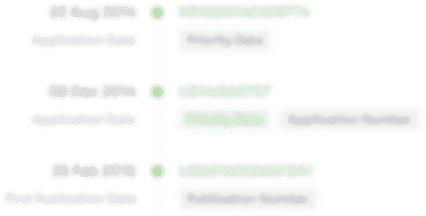
- R&D Engineer
- R&D Manager
- IP Professional
- Industry Leading Data Capabilities
- Powerful AI technology
- Patent DNA Extraction
Browse by: Latest US Patents, China's latest patents, Technical Efficacy Thesaurus, Application Domain, Technology Topic.
© 2024 PatSnap. All rights reserved.Legal|Privacy policy|Modern Slavery Act Transparency Statement|Sitemap