Equipment fault self-adaptive upper and lower early warning boundary generation method based on convolutional neural network
A convolutional neural network and equipment failure technology, applied in neural learning methods, biological neural network models, neural architectures, etc., can solve problems such as low accuracy of fault diagnosis technology and being easily affected by human factors
- Summary
- Abstract
- Description
- Claims
- Application Information
AI Technical Summary
Problems solved by technology
Method used
Image
Examples
Embodiment Construction
[0061] Below in conjunction with specific embodiment, further illustrate the present invention. It should be understood that these examples are only used to illustrate the present invention and are not intended to limit the scope of the present invention. In addition, it should be understood that after reading the teachings of the present invention, those skilled in the art can make various changes or modifications to the present invention, and these equivalent forms also fall within the scope defined by the appended claims of the present application.
[0062] The invention provides a method for generating adaptive upper and lower early warning boundaries for equipment faults based on a convolutional neural network, comprising the following steps:
[0063] Step 1. Collect the vibration signal during the operation of the chuck of the chemical fiber winding machine, and store the original signal in the computer by using an acceleration sensor and a collection card. And the coll...
PUM
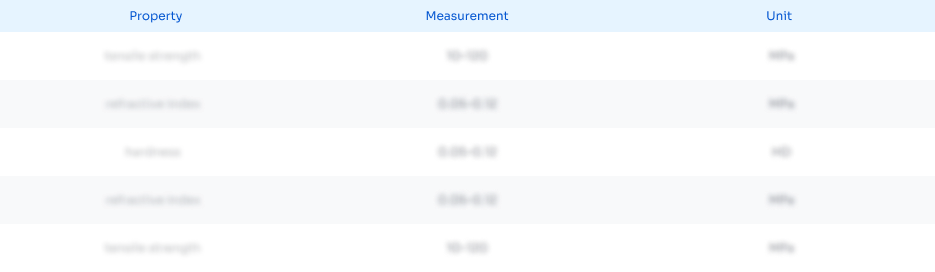
Abstract
Description
Claims
Application Information
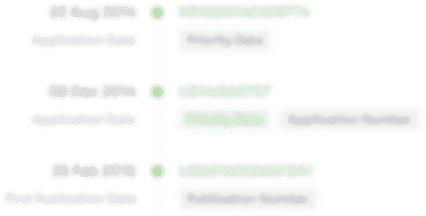
- R&D Engineer
- R&D Manager
- IP Professional
- Industry Leading Data Capabilities
- Powerful AI technology
- Patent DNA Extraction
Browse by: Latest US Patents, China's latest patents, Technical Efficacy Thesaurus, Application Domain, Technology Topic.
© 2024 PatSnap. All rights reserved.Legal|Privacy policy|Modern Slavery Act Transparency Statement|Sitemap