Swarm optimization kernel extreme learning and sparse representation mechanical fault identification method
A nuclear extreme learning machine and fault identification technology, which is applied in neural learning methods, character and pattern recognition, design optimization/simulation, etc., can solve the problems that are difficult to meet actual requirements, mechanical fault identification methods are difficult to meet the requirements of actual production, and classification efficiency low level problem
- Summary
- Abstract
- Description
- Claims
- Application Information
AI Technical Summary
Problems solved by technology
Method used
Image
Examples
Embodiment Construction
[0043] The present invention will be described in further detail below in conjunction with accompanying drawing and embodiment, described is the explanation of the present invention rather than limitation.
[0044] The present invention uses the nuclear extreme learning machine fault identification method to identify the input mechanical vibration signal, and integrates the artificial bee colony optimization algorithm (ABC) into the nuclear extreme learning method, and obtains the nuclear extreme learning machine through the artificial bee colony optimization algorithm (ABC). The optimal model parameters of the fault identification model are used to improve the performance of the fault identification model of the nuclear extreme learning machine. According to the fault identification results of the bee colony optimization nuclear extreme learning machine method, if the identification results do not reach the preset threshold effect, then the sparse representation is used The me...
PUM
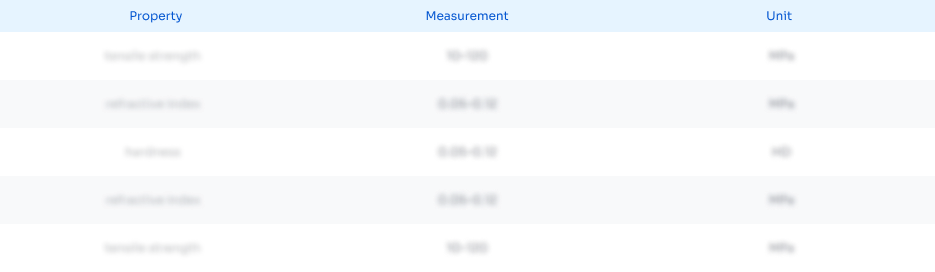
Abstract
Description
Claims
Application Information
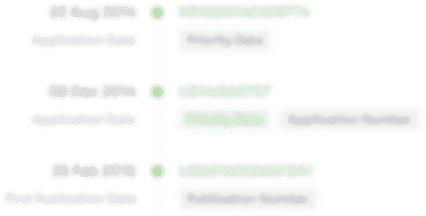
- R&D Engineer
- R&D Manager
- IP Professional
- Industry Leading Data Capabilities
- Powerful AI technology
- Patent DNA Extraction
Browse by: Latest US Patents, China's latest patents, Technical Efficacy Thesaurus, Application Domain, Technology Topic.
© 2024 PatSnap. All rights reserved.Legal|Privacy policy|Modern Slavery Act Transparency Statement|Sitemap