Valve-regulated lead-acid storage battery health state prediction method based on improved LSTM neural network
A lead-acid battery, neural network technology, applied in the field of artificial intelligence control of valve-regulated lead-acid batteries in substations, can solve problems such as excessive consumption and environmental pollution, achieve high accuracy, reduce dependence, and improve accuracy.
- Summary
- Abstract
- Description
- Claims
- Application Information
AI Technical Summary
Problems solved by technology
Method used
Image
Examples
Embodiment
[0082] see figure 1 , LSTM-based network training, the specific contents include:
[0083] S11. Calculate the connection strength R of neuron i i (t). When the error between the model prediction value and the real value is less than the set threshold, the connection strength is calculated by formula (8).
[0084] S12, update the activation state S of the neuron in the iterative process according to formula (9) i (t+1). That is, neurons with higher connection strength have a greater probability of transitioning to an inactive state. In this way, the dependence of the LSTM prediction model on some input features is reduced.
[0085] see figure 2 , the network training of LSTM based on the Dropout optimization method, the specific contents include:
[0086] S21, LSTM network initialization. Given the number of input nodes m, the number of hidden nodes k, the number of output nodes n, the learning rate yita, and the error threshold σ, specify the dimensions of each weight...
PUM
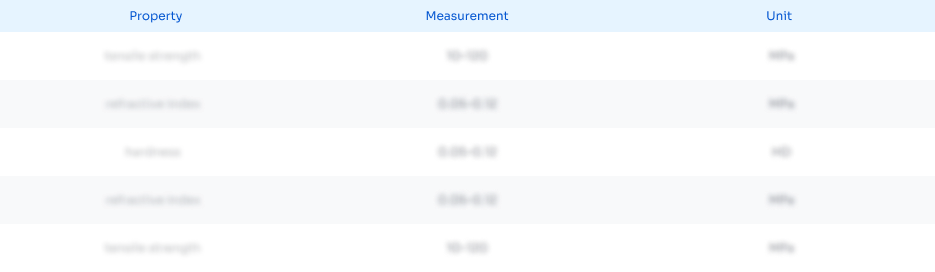
Abstract
Description
Claims
Application Information
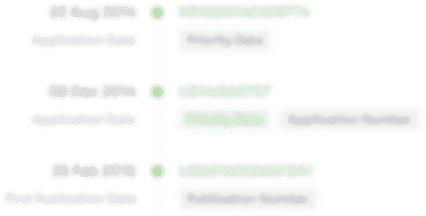
- R&D Engineer
- R&D Manager
- IP Professional
- Industry Leading Data Capabilities
- Powerful AI technology
- Patent DNA Extraction
Browse by: Latest US Patents, China's latest patents, Technical Efficacy Thesaurus, Application Domain, Technology Topic, Popular Technical Reports.
© 2024 PatSnap. All rights reserved.Legal|Privacy policy|Modern Slavery Act Transparency Statement|Sitemap|About US| Contact US: help@patsnap.com