Distributed machine learning system acceleration method based on network reconfiguration
A network acceleration and machine learning technology, applied in neural learning methods, biological neural network models, instruments, etc., can solve the problem of not fully considering the characteristics of machine learning task load, long tail delay and other problems, to ensure efficient operation, guarantee The effect of fair distribution and avoiding long tail delay
- Summary
- Abstract
- Description
- Claims
- Application Information
AI Technical Summary
Problems solved by technology
Method used
Image
Examples
Embodiment Construction
[0030] The present invention will be further described below in conjunction with accompanying drawing, please refer to figure 1 and image 3 ; figure 1 The architecture of the method for improving the distributed training speed of machine learning models based on network reconfiguration proposed by the present invention is given. Among them, 1 is the model database; 2, 3, and 4 are the scheduling policy manager, scheduler, and state memory, which constitute the resource coordinator; 5, 6, and 7 are the wireless router on the top of the rack and the working machine inside the rack. and switches.
[0031] The important components of the system structure of the present invention will be described in detail below.
[0032] (1) Model database
[0033] The model database is used to store the machine learning model to be trained submitted by the user, and to store the relevant parameters of the model to be trained. The resource coordinator will actively pull the model to be train...
PUM
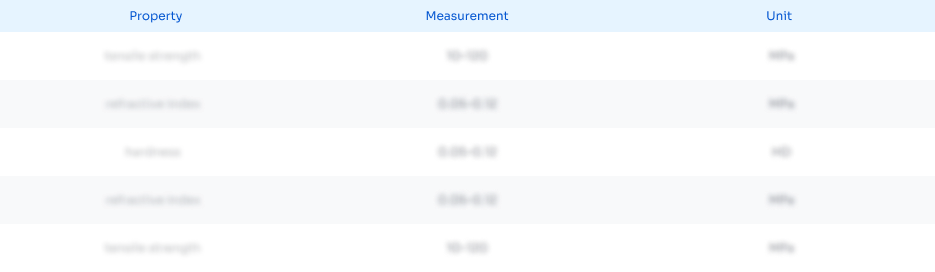
Abstract
Description
Claims
Application Information
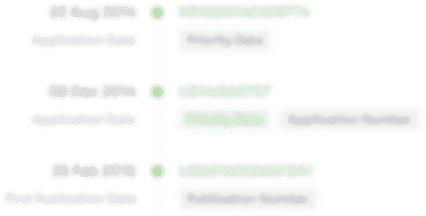
- R&D
- Intellectual Property
- Life Sciences
- Materials
- Tech Scout
- Unparalleled Data Quality
- Higher Quality Content
- 60% Fewer Hallucinations
Browse by: Latest US Patents, China's latest patents, Technical Efficacy Thesaurus, Application Domain, Technology Topic, Popular Technical Reports.
© 2025 PatSnap. All rights reserved.Legal|Privacy policy|Modern Slavery Act Transparency Statement|Sitemap|About US| Contact US: help@patsnap.com