Photovoltaic field station generated power prediction method and system
A technology of power generation and forecasting methods, applied in forecasting, information technology support systems, instruments, etc., can solve problems such as uncertainty, discontinuity of output power, and failure to consider the impact of random fluctuations in power generation
- Summary
- Abstract
- Description
- Claims
- Application Information
AI Technical Summary
Problems solved by technology
Method used
Image
Examples
Embodiment 1
[0035] In this embodiment, integrated learning is applied to the power generation prediction of photovoltaic plants and stations. Integrated learning is a combination method, and the performance is better than that of a single learner by combining a variety of different learners; Then combine them, and then train the integrated model, usually using existing learning algorithms, such as decision trees, neural networks, support vector machines, etc., to generate independent learners from the training data. Compared with a single model, integrated learning can fully mine the information in training samples, so as to obtain more accurate and reliable prediction results.
[0036] like figure 1 As shown, this embodiment provides a method for predicting power generation of a photovoltaic field station, including:
[0037] S1: Construct a training sample set according to the obtained historical power data of the photovoltaic station and the meteorological data of the corresponding ti...
Embodiment 2
[0079] This embodiment provides a photovoltaic power generation power prediction system, including:
[0080] The weight allocation module is used to construct a training sample set according to the acquired historical power data of the photovoltaic station and the meteorological data of the corresponding time period, and allocate a sample weight to each training sample in the training sample set;
[0081] The training module is used to train the random forest model using the sub-training sample set generated by the self-service sampling method for the training sample set, and calculate the error rate and weight coefficient of the random forest model under the current sample weight according to the adaptive enhancement algorithm;
[0082] The iteration module is used to update the sample weight according to the error rate and the weight coefficient under the preset number of iterations, train the random forest model in turn, and weight it according to the weight coefficient to o...
PUM
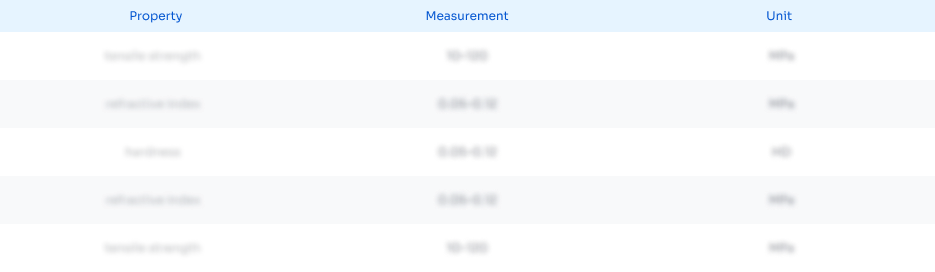
Abstract
Description
Claims
Application Information
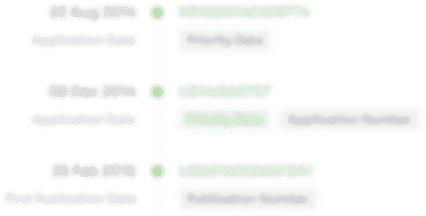
- R&D
- Intellectual Property
- Life Sciences
- Materials
- Tech Scout
- Unparalleled Data Quality
- Higher Quality Content
- 60% Fewer Hallucinations
Browse by: Latest US Patents, China's latest patents, Technical Efficacy Thesaurus, Application Domain, Technology Topic, Popular Technical Reports.
© 2025 PatSnap. All rights reserved.Legal|Privacy policy|Modern Slavery Act Transparency Statement|Sitemap|About US| Contact US: help@patsnap.com