Textile defect detection method based on low-rank sparse matrix decomposition
A sparse matrix and defect detection technology, applied in image analysis, image data processing, instruments, etc., can solve problems such as separation and failure to detect uniform defect blocks
- Summary
- Abstract
- Description
- Claims
- Application Information
AI Technical Summary
Problems solved by technology
Method used
Image
Examples
Embodiment 1
[0030] Such as figure 1 As shown, a textile defect detection method based on low-rank sparse matrix factorization, including the following steps:
[0031] S1: Input a flawless textile image with periodically changing patterns;
[0032] S2: Determine the size of the pattern period template in the textile image, divide the flawless textile image into blocks according to the size of the pattern period template, and obtain multiple training feature blocks; specifically, the size of each feature block is the same as the pattern period template. ;
[0033] S3: Extract the Gabor feature of each training feature block, calculate the Chebyshev distance between the training feature blocks, and construct a feature distance matrix;
[0034] In this step, the Gabor filter is used to extract the Gabor feature of each training feature block, and the Gabor filter bank defining the two-dimensional Gabor transformation function is as follows:
[0035]
[0036]
[0037] Among them, the pa...
PUM
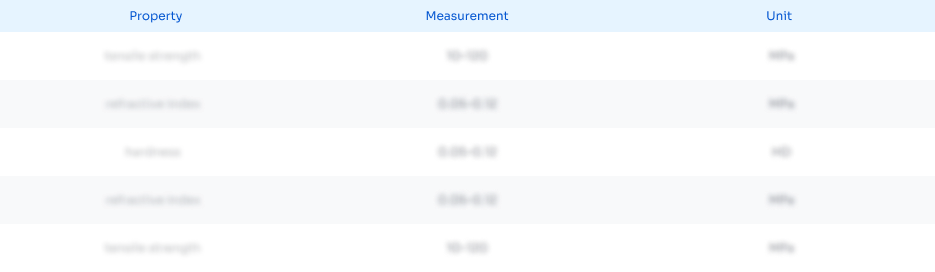
Abstract
Description
Claims
Application Information
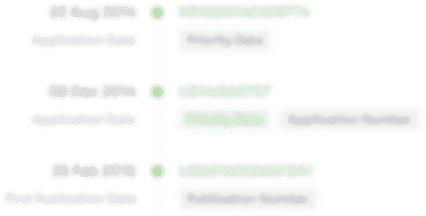
- R&D
- Intellectual Property
- Life Sciences
- Materials
- Tech Scout
- Unparalleled Data Quality
- Higher Quality Content
- 60% Fewer Hallucinations
Browse by: Latest US Patents, China's latest patents, Technical Efficacy Thesaurus, Application Domain, Technology Topic, Popular Technical Reports.
© 2025 PatSnap. All rights reserved.Legal|Privacy policy|Modern Slavery Act Transparency Statement|Sitemap|About US| Contact US: help@patsnap.com