Monitoring data fault detection method and system
A technology for monitoring data and fault detection, applied in digital data information retrieval, electrical digital data processing, special data processing applications, etc., can solve problems such as ignoring local structure information of different data points
- Summary
- Abstract
- Description
- Claims
- Application Information
AI Technical Summary
Problems solved by technology
Method used
Image
Examples
Embodiment 1
[0066] see figure 1 , is a flow chart of the monitoring data fault detection method provided by the first embodiment of the present invention, including steps:
[0067] Step S10, performing standardized processing on the monitoring data to be tested;
[0068] Specifically, in this step, the step of standardizing the monitoring data to be tested includes:
[0069] Obtain each variable in the monitoring data to be tested;
[0070] Subtracting the variable from the mean value obtained in the corresponding training data to obtain the target difference;
[0071] Dividing the target difference by the variance obtained in the training data completes the standard process.
[0072] Step S20, sampling the monitoring data to be tested to obtain sampled data;
[0073] Step S30, construct past vector and future vector according to described sampling data, and calculate past kernel matrix and future kernel matrix about described sampling data;
[0074] Step S40, standardize the past ke...
Embodiment 2
[0078] see figure 2 , is a flowchart of the monitoring data fault detection method provided in the second embodiment of the present invention, including steps:
[0079] Step S11, obtain dimensionless data, respectively select the first lag and the second lag to construct past vectors and future vectors, and construct past Hankel matrix and future Hankel matrix;
[0080] Step S21, selecting a radial basis kernel function, and calculating a past kernel matrix and a future kernel matrix;
[0081] Carry out matrix calculation to described past kernel matrix and described future kernel matrix respectively, to obtain the first Laplacian matrix and the second Laplacian matrix;
[0082] Step S31, calculating the parameter values of α and β according to the optimization formula;
[0083] Specifically, this embodiment proposes a fault detection method based on Local Kernel Canonical Variable Analysis (LPP-KCVA), which takes into account local structure information and non-local str...
Embodiment 3
[0133] see Figure 19 , is a schematic structural diagram of the monitoring data fault detection system 100 provided in the third embodiment of the present invention, wherein:
[0134] The data sampling module 11 carries out standardized processing to the monitoring data to be measured, and samples the monitoring data to be measured to obtain sampling data;
[0135] Matrix calculation module 12, for constructing past vector and future vector according to described sampling data, and calculate past kernel matrix and future kernel matrix about described sampling data;
[0136] Statistic calculation module 13, used to carry out standardization process to described kernel matrix in the past and described future kernel matrix, and calculate the corresponding statistic with state space and residual space according to preset off-line model;
[0137] Fault judgment module 14, is used for comparing described statistic with preset control limit, when judging that described statistic is...
PUM
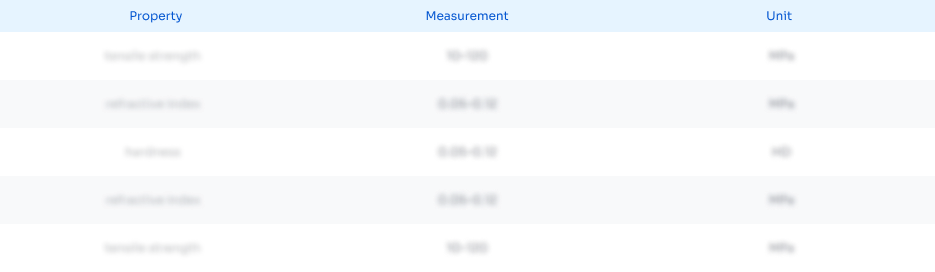
Abstract
Description
Claims
Application Information
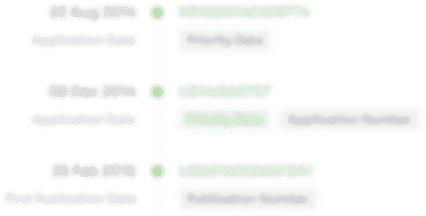
- Generate Ideas
- Intellectual Property
- Life Sciences
- Materials
- Tech Scout
- Unparalleled Data Quality
- Higher Quality Content
- 60% Fewer Hallucinations
Browse by: Latest US Patents, China's latest patents, Technical Efficacy Thesaurus, Application Domain, Technology Topic, Popular Technical Reports.
© 2025 PatSnap. All rights reserved.Legal|Privacy policy|Modern Slavery Act Transparency Statement|Sitemap|About US| Contact US: help@patsnap.com