Unsupervised enhancement method for surface defect image data of hot-rolled plate coil
An image data and hot-rolled coil technology, which is applied in the field of image data generation of hot-rolled coil surface defect image data based on artificial intelligence network model, can solve the problem of affecting product quality, less picture data, and low accuracy of defect recognition by artificial intelligence machine vision technology. And other issues
- Summary
- Abstract
- Description
- Claims
- Application Information
AI Technical Summary
Problems solved by technology
Method used
Image
Examples
Embodiment Construction
[0043] 1. The implementation of this method requires a computer server for deep learning, 2 GPU graphics cards of Tesla (version V100), each with a memory size of 32G, 128 internal memory, and 1TB solid-state hard drive as the hardware platform;
[0044] 2. Install the Linux 64-bit (Ubuntu16.04) operating system on the computer, install the Anaconda software library, install the Pytorch deep learning framework, install the CUDAToolkit version 10.2, the graphics driver version is NVIDI-440.33.01, and the cudnn acceleration package version is 7.6.5 As a software environment, use python programming language;
[0045] 3. Use the command statement source axtivatepytorch to activate the development environment for deep learning;
[0046] 4. Prepare the data set. The picture training set in the present invention comes from the actual production line of hot-rolled coils in the steel mill, and is photographed and collected by the surface defect detector. The labeled data set after work...
PUM
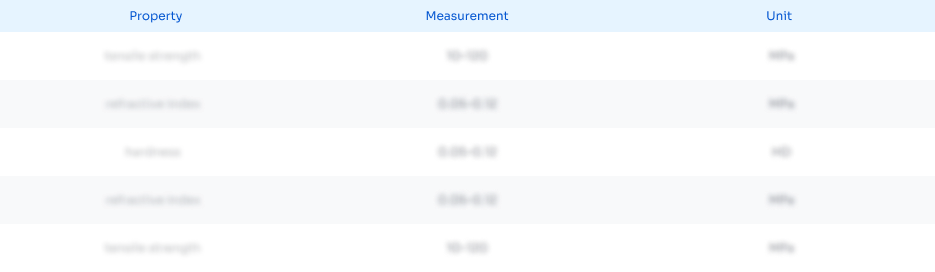
Abstract
Description
Claims
Application Information
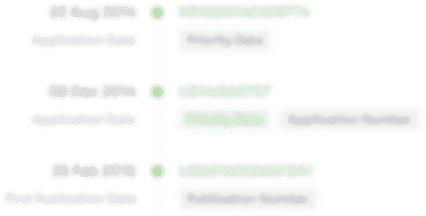
- R&D
- Intellectual Property
- Life Sciences
- Materials
- Tech Scout
- Unparalleled Data Quality
- Higher Quality Content
- 60% Fewer Hallucinations
Browse by: Latest US Patents, China's latest patents, Technical Efficacy Thesaurus, Application Domain, Technology Topic, Popular Technical Reports.
© 2025 PatSnap. All rights reserved.Legal|Privacy policy|Modern Slavery Act Transparency Statement|Sitemap|About US| Contact US: help@patsnap.com