Electrocardiogram anomaly recognition method and system based on combination of AlexNet and transfer learning
A technology of transfer learning and abnormal identification, applied in the field of electrocardiogram abnormal identification method and system, to achieve the effect of reducing the amount of parameters and reducing over-fitting
- Summary
- Abstract
- Description
- Claims
- Application Information
AI Technical Summary
Problems solved by technology
Method used
Image
Examples
Embodiment 1
[0108] as attached figure 1 As shown, the ECG abnormal recognition method based on AlexNet and migration learning of the present invention is combined, and the method is specifically as follows:
[0109] S1. Preprocessing: Convert each ECG signal in the data set into an ECG image, and cut out abnormal ECGs in different directions to achieve the purpose of data enhancement; the details are as follows:
[0110] S101. Image conversion: converting each ECG signal in the data set into an ECG image with a size of 227x227;
[0111] S102. Construct heartbeat samples: use wavelet transform to process high-frequency signals of power frequency signal interference and myoelectric signal interference; IIR zero-phase digital filter corrects baseline drift, and realizes QRS wave detection by software discrimination and variable threshold; After the QRS wave is detected, the ECG is segmented around the QRS wave peak, and a total of 256 samples are taken from 90 samples before the QRS wave pe...
Embodiment 2
[0142] The electrocardiogram abnormal recognition system based on the combination of AlexNet and transfer learning of the present invention, the system includes,
[0143] The preprocessing unit is used to convert each electrocardiogram signal in the data set into an electrocardiogram image, and clip abnormal types of electrocardiograms in different directions to achieve the purpose of data enhancement; the preprocessing unit includes,
[0144] The image conversion module is used to convert each electrocardiogram signal in the data set into an electrocardiogram image whose size is 227x227;
[0145] Heart beat sample building module, used to segment ECG around the QRS peak, taking 90 samples before the QRS peak and 165 samples after the peak, a total of 256 samples to form a heart beat sample;
[0146] The ECG amplitude influence module is used to normalize the heartbeat sample on the basis of dividing the heartbeat sample, and eliminate the influence of different amplitudes in ...
Embodiment 3
[0169] The embodiment of the present invention also provides a computer-readable storage medium, in which a plurality of instructions are stored, and the instructions are loaded by the processor, so that the processor executes the abnormal electrocardiogram based on the combination of AlexNet and migration learning in any embodiment of the present invention recognition methods. Specifically, a system or device equipped with a storage medium may be provided, on which a software program code for realizing the functions of any of the above embodiments is stored, and the computer (or CPU or MPU of the system or device) ) to read and execute the program code stored in the storage medium.
PUM
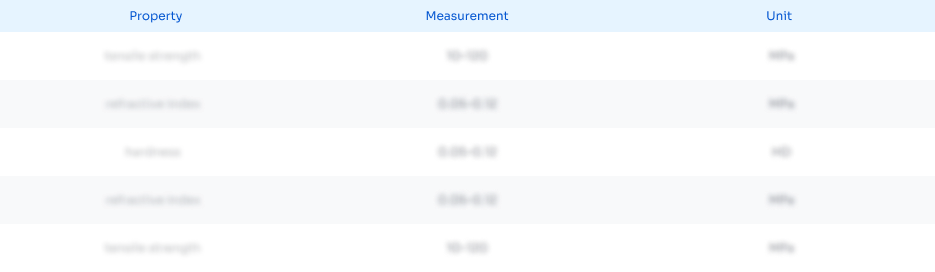
Abstract
Description
Claims
Application Information
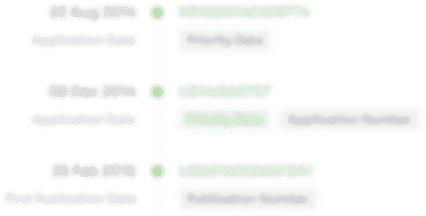
- R&D Engineer
- R&D Manager
- IP Professional
- Industry Leading Data Capabilities
- Powerful AI technology
- Patent DNA Extraction
Browse by: Latest US Patents, China's latest patents, Technical Efficacy Thesaurus, Application Domain, Technology Topic, Popular Technical Reports.
© 2024 PatSnap. All rights reserved.Legal|Privacy policy|Modern Slavery Act Transparency Statement|Sitemap|About US| Contact US: help@patsnap.com