Motor bearing fault diagnosis method based on generalized S transformation and WOA-SVM
A technology for motor bearing and fault diagnosis, which is applied in the testing of computer components and mechanical components, character and pattern recognition, etc., can solve the problems of limited performance of SVM classification, improve recognition accuracy, increase correct rate, and adjust parameters little effect
- Summary
- Abstract
- Description
- Claims
- Application Information
AI Technical Summary
Problems solved by technology
Method used
Image
Examples
Embodiment Construction
[0063] Such as figure 1 As shown, a motor bearing fault diagnosis method based on generalized S-transform and WOA-SVM, the method includes the following sequential steps:
[0064] (1) Input the vibration signal of the motor bearing, and obtain two time-frequency matrices after two different generalized S transformations;
[0065] (2) According to the two time-frequency matrices, the time-domain cumulative characteristic curve with high time resolution and the frequency-domain cumulative characteristic curve with high frequency resolution are respectively obtained;
[0066] (3) Extract the mean value and standard deviation of the amplitudes of the time-domain cumulative characteristic curve and the frequency-domain cumulative characteristic curve, respectively, to obtain the time-domain characteristics and frequency-domain characteristics of the original signal;
[0067] (4) Combining time-domain features and frequency-domain features to form a feature vector sample set, which...
PUM
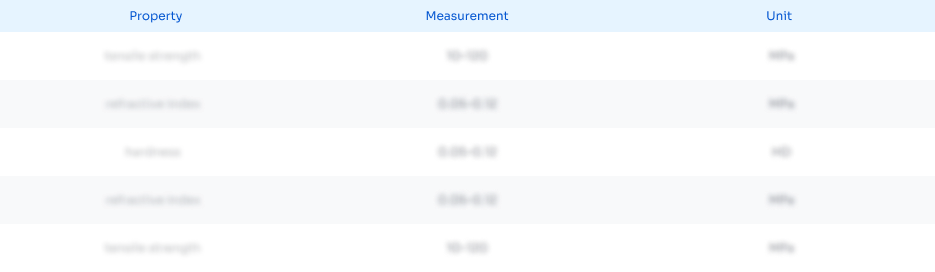
Abstract
Description
Claims
Application Information
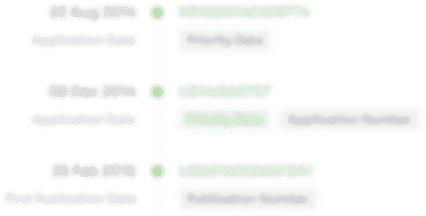
- R&D Engineer
- R&D Manager
- IP Professional
- Industry Leading Data Capabilities
- Powerful AI technology
- Patent DNA Extraction
Browse by: Latest US Patents, China's latest patents, Technical Efficacy Thesaurus, Application Domain, Technology Topic, Popular Technical Reports.
© 2024 PatSnap. All rights reserved.Legal|Privacy policy|Modern Slavery Act Transparency Statement|Sitemap|About US| Contact US: help@patsnap.com