Digestive endoscopy video scene classification method based on convolutional neural network
A convolutional neural network and digestive endoscopy technology, applied in biological neural network models, endoscopy, neural architecture, etc., can solve single-frame misclassification, need to start the identification of intestinal polyps, and single-frame image scene misjudgment and other problems to achieve the effect of enhancing reliability
- Summary
- Abstract
- Description
- Claims
- Application Information
AI Technical Summary
Problems solved by technology
Method used
Image
Examples
Embodiment Construction
[0046] The present invention will be further described in detail below in conjunction with the accompanying drawings and specific embodiments.
[0047] Please refer to the attached figure 1 A method for classifying scenes of digestive endoscopy videos based on convolutional neural networks according to the present invention is to solve the problem of automatic recognition of scenes in digestive endoscopy videos in AI-based digestive endoscopy CAD systems, and includes the following steps :
[0048] (1): Obtain real-time scene images through digestive endoscopy equipment.
[0049] (2): Use the CNN scene classifier to perform preliminary scene classification on the acquired scene images.
[0050] (3): Incorporate the preliminary classification results of the CNN scene classifier into the K sliding window timing signal queue, where K is the length of the timing signal queue.
[0051] (4): Count the proportions of various states in the K sliding window queue.
[0052] (5): Det...
PUM
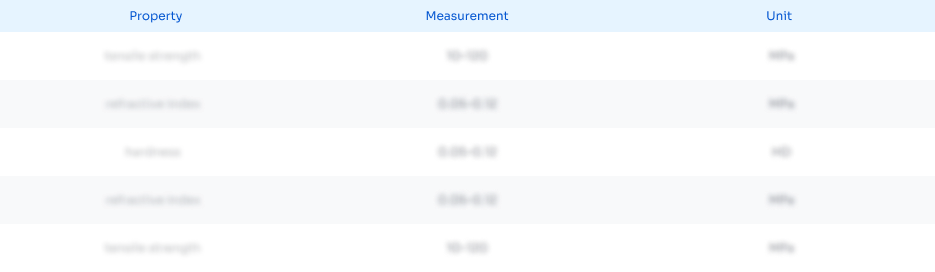
Abstract
Description
Claims
Application Information
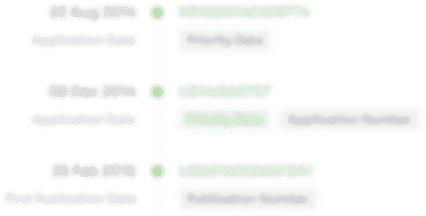
- R&D
- Intellectual Property
- Life Sciences
- Materials
- Tech Scout
- Unparalleled Data Quality
- Higher Quality Content
- 60% Fewer Hallucinations
Browse by: Latest US Patents, China's latest patents, Technical Efficacy Thesaurus, Application Domain, Technology Topic, Popular Technical Reports.
© 2025 PatSnap. All rights reserved.Legal|Privacy policy|Modern Slavery Act Transparency Statement|Sitemap|About US| Contact US: help@patsnap.com