Multi-label rolling bearing fault diagnosis method based on meta-learning
A rolling bearing and fault diagnosis technology, which is applied in the field of multi-label rolling bearing fault diagnosis based on meta-learning, can solve the problems of unfavorable rolling bearing fault diagnosis, sensitive fault features, small sample fault diagnosis accuracy to be further improved, etc.
- Summary
- Abstract
- Description
- Claims
- Application Information
AI Technical Summary
Problems solved by technology
Method used
Image
Examples
Embodiment Construction
[0125] Below in conjunction with accompanying drawing, further describe the present invention through embodiment, but do not limit the scope of the present invention in any way.
[0126] The following embodiments use the vibration data of rolling bearings measured by the Bearing Data Center of Case Western Reserve University to describe in detail the implementation process of the diagnosis method provided by the present invention.
[0127] Method flow chart as figure 2 shown. The method of the invention includes: 1) constructing a multi-label fault data set of rolling bearings and dividing it into a training set and a test set according to the fault category; 2) extracting the time-frequency signature matrix feature T-FSMs of the fault signal; 3) establishing a meta-learning Functional multi-label convolutional neural network model MLCML; 4) Use the training set samples to train the MLCML model; 5) Use the test set samples to verify the MLCML model, and apply the model to th...
PUM
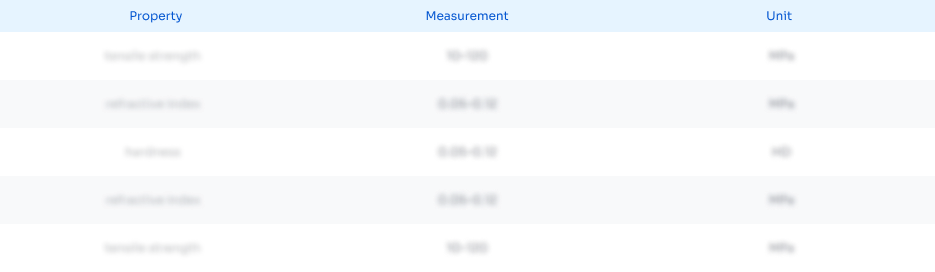
Abstract
Description
Claims
Application Information
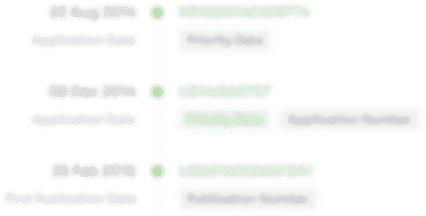
- R&D Engineer
- R&D Manager
- IP Professional
- Industry Leading Data Capabilities
- Powerful AI technology
- Patent DNA Extraction
Browse by: Latest US Patents, China's latest patents, Technical Efficacy Thesaurus, Application Domain, Technology Topic, Popular Technical Reports.
© 2024 PatSnap. All rights reserved.Legal|Privacy policy|Modern Slavery Act Transparency Statement|Sitemap|About US| Contact US: help@patsnap.com