Hematoxylin-eosin staining pathological image segmentation method based on unsupervised deep learning
A deep learning and pathological image technology, applied in the field of image processing, can solve the problems of differences in staining results, poor generalization ability, and difficulty in establishing a stable shape model, and achieve high precision and efficiency, high deep learning precision, and improved production efficiency. Effect
- Summary
- Abstract
- Description
- Claims
- Application Information
AI Technical Summary
Problems solved by technology
Method used
Image
Examples
Embodiment Construction
[0051] In order to make the purpose, technical solutions and advantages of the embodiments of the present application clearer, the technical solutions in the embodiments of the present application will be clearly and completely described below in conjunction with the drawings in the embodiments of the present application.
[0052] Such as Figures 1 to 5 As shown in one of them, the present invention discloses a hematoxylin-eosin staining pathological image segmentation method based on unsupervised deep learning, which comprises the following steps:
[0053] Step 1. Feature extraction is performed on hematoxylin-eosin (HE) stained pathological images, including steps such as filtering, obtaining reliable class labels, and calculating mutual information. HE stained pathological images were preprocessed by combining Gaussian filter with a window size of 5×5 and median filter, and feature selection was performed in RGB color space based on mutual information results to obtain a s...
PUM
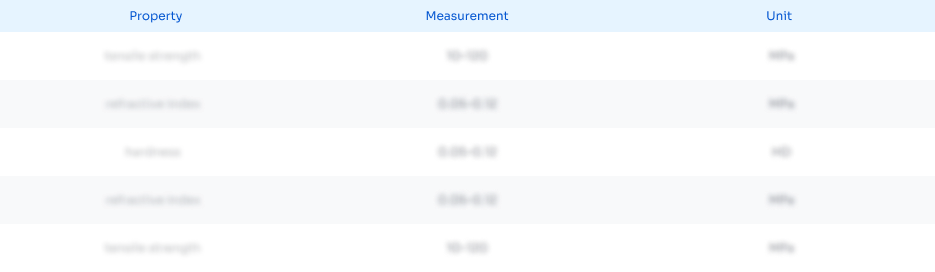
Abstract
Description
Claims
Application Information
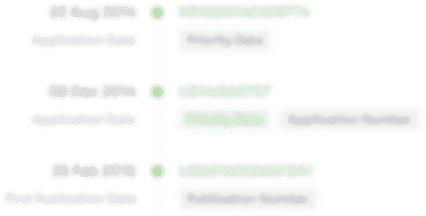
- R&D Engineer
- R&D Manager
- IP Professional
- Industry Leading Data Capabilities
- Powerful AI technology
- Patent DNA Extraction
Browse by: Latest US Patents, China's latest patents, Technical Efficacy Thesaurus, Application Domain, Technology Topic, Popular Technical Reports.
© 2024 PatSnap. All rights reserved.Legal|Privacy policy|Modern Slavery Act Transparency Statement|Sitemap|About US| Contact US: help@patsnap.com