Spectral domain graph convolution 3D point cloud classification method based on Fourier transform
A technology of Fourier transform and classification method, which is applied in image analysis, neural learning methods, image enhancement, etc., can solve the problems of lack, uneven distribution of point cloud density, etc., and achieve the effect of strong anti-noise ability and high calculation efficiency
- Summary
- Abstract
- Description
- Claims
- Application Information
AI Technical Summary
Problems solved by technology
Method used
Image
Examples
Embodiment Construction
[0028] Embodiments of the present invention will be described below in conjunction with the accompanying drawings.
[0029] Such as figure 1 As shown, the present invention designs a 3D point cloud classification method based on Fourier transform-based spectral domain graph convolution. The process can be divided into three parts: geometric sampling, Dynamic KNN local graph construction, and Fourier transform-based spectral domain graph convolution. , including the following steps:
[0030] Step 1. Use the G-PointNet network model to perform geometric sampling processing on the input original point cloud. details as follows:
[0031] First, the G-PointNet network model of the present invention is obtained under the inspiration of the PointNet deep network model and the spectral domain graph convolution operation based on Fourier transform. The G-PointNet network model retains the spatial transformation network T-Net in PointNet. The G-PointNet network model directly uses p...
PUM
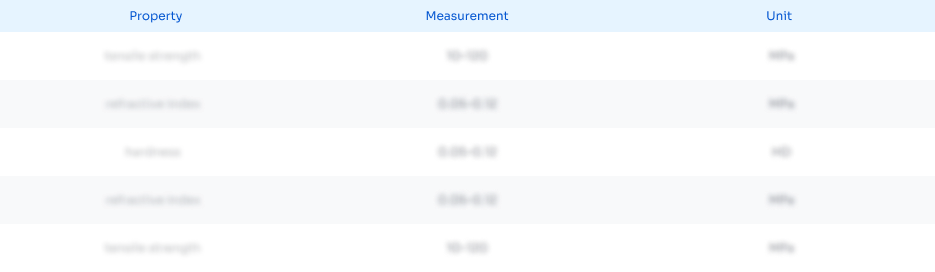
Abstract
Description
Claims
Application Information
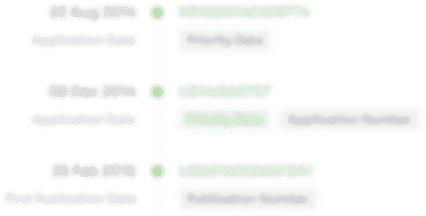
- R&D
- Intellectual Property
- Life Sciences
- Materials
- Tech Scout
- Unparalleled Data Quality
- Higher Quality Content
- 60% Fewer Hallucinations
Browse by: Latest US Patents, China's latest patents, Technical Efficacy Thesaurus, Application Domain, Technology Topic, Popular Technical Reports.
© 2025 PatSnap. All rights reserved.Legal|Privacy policy|Modern Slavery Act Transparency Statement|Sitemap|About US| Contact US: help@patsnap.com