Electrocardiogram identity recognition method
A technology of identity recognition and electrocardiogram signal, which is applied in the field of identity recognition, can solve problems such as increased computational burden, difficulty in classifying targets, and impact on recognition accuracy, so as to achieve accurate and error-free identity recognition and avoid complexity
- Summary
- Abstract
- Description
- Claims
- Application Information
AI Technical Summary
Problems solved by technology
Method used
Image
Examples
Embodiment
[0071] Embodiment: a kind of ECG identification method of this embodiment, such as figure 1 , figure 2 , image 3 shown, including the following steps:
[0072] S1: collect the original ECG signal of the human body;
[0073] S2: Preprocessing the original ECG signal to obtain a short-period ECG signal;
[0074] S3: Perform generalized S-transformation on the short-period ECG signal to extract the phase-domain feature vector Y 1 , time-domain feature vector Y 2 , frequency domain feature vector Y 3 ;
[0075] S4: The phase domain feature vector Y 1 , time-domain feature vector Y 2 , frequency domain feature vector Y 3 Input the nonlinear approximation model of sparsity constraints, obtain the optimal dictionary and the corresponding optimal sparse coefficient matrix, and perform lightweight processing on the optimal sparse coefficient matrix to obtain the sparse coefficient vector;
[0076] S5: Input the sparse coefficient vector into the trained deep neural network ...
PUM
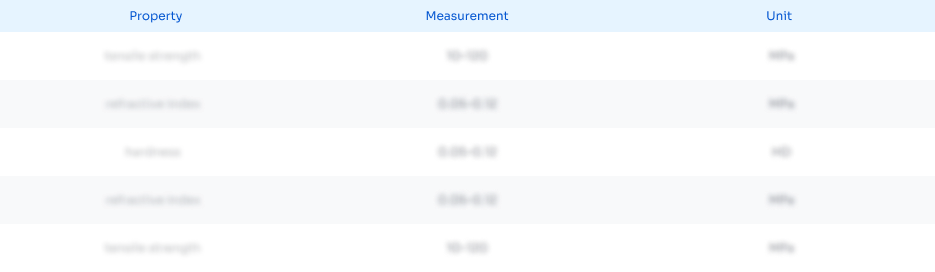
Abstract
Description
Claims
Application Information
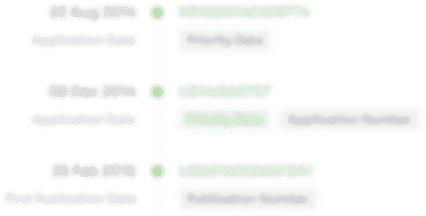
- R&D
- Intellectual Property
- Life Sciences
- Materials
- Tech Scout
- Unparalleled Data Quality
- Higher Quality Content
- 60% Fewer Hallucinations
Browse by: Latest US Patents, China's latest patents, Technical Efficacy Thesaurus, Application Domain, Technology Topic, Popular Technical Reports.
© 2025 PatSnap. All rights reserved.Legal|Privacy policy|Modern Slavery Act Transparency Statement|Sitemap|About US| Contact US: help@patsnap.com