Skull image segmentation method based on deep iterative fusion deep learning model
A deep learning and image segmentation technology, applied in the field of medical image processing and deep learning, can solve the problems of generalization ability, slow training time, poor performance, etc., to enhance trainability, improve accuracy, and improve cognition Effect
- Summary
- Abstract
- Description
- Claims
- Application Information
AI Technical Summary
Problems solved by technology
Method used
Image
Examples
Embodiment 1
[0022]Example 1: As attachedFigure 1~4As shown, a skull image segmentation method based on deep iterative fusion deep learning model includes the following steps: 1. Select the brain magnetic resonance image that needs to be segmented and preprocess it; 2. Preprocess Randomly select the training set and test set for the later brain magnetic resonance images; third, build a deep iterative fusion learning model, which includes a residual module and an up-sampling module; fourth, enhance the internal data of the training set, including random scaling And random cropping; Fifth, the data training model after data enhancement, and finally use the trained model to test the test set.
[0023]In the first step, the method for preprocessing the brain magnetic resonance image is:
[0024]
[0025]Where xiMeans the input image, min(x), max(x) means the minimum and maximum value of the input image, xkIs the normalized data.
[0026]In the second step, the division method is: suppose the total number of ind...
PUM
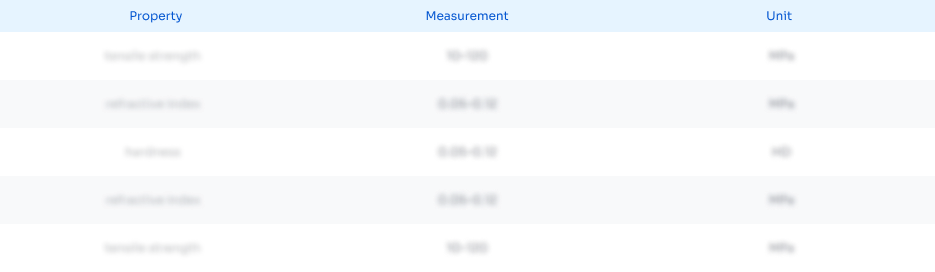
Abstract
Description
Claims
Application Information
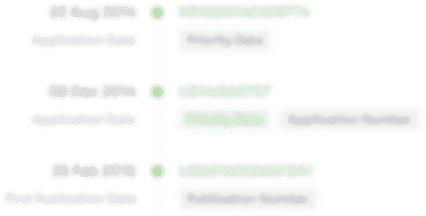
- R&D Engineer
- R&D Manager
- IP Professional
- Industry Leading Data Capabilities
- Powerful AI technology
- Patent DNA Extraction
Browse by: Latest US Patents, China's latest patents, Technical Efficacy Thesaurus, Application Domain, Technology Topic, Popular Technical Reports.
© 2024 PatSnap. All rights reserved.Legal|Privacy policy|Modern Slavery Act Transparency Statement|Sitemap|About US| Contact US: help@patsnap.com