Imperceptible adversarial patch generation method and application
A patch, adversarial sample technology, applied in neural learning methods, biological neural network models, instruments, etc., can solve problems such as deception recognition systems, less training to enhance model defense capabilities, and no consideration of patch imperceptibility.
- Summary
- Abstract
- Description
- Claims
- Application Information
AI Technical Summary
Problems solved by technology
Method used
Image
Examples
Embodiment 1
[0119] 1. The present invention attempts to propose a new method of adversarial patching, which attempts to use the interpretability of CNNs to create patch adversarial samples at the semantic level, with the purpose of conducting adversarial training and enhancing the defense capabilities of deep neural networks. The method combines Grad-CAM and optimization techniques to achieve a good balance between adversarial strength and imperceptibility. The present invention improves the adversarial patch method in two aspects: first, the magnitude of the perturbation is greatly reduced, which is almost imperceptible to humans; second, the size of the adversarial patch is significantly reduced.
[0120] 2. The present invention proves the reasonable explanation of Grad-CAM through adversarial examples. The present work shows that adversarial perturbations in CFRs localized by Grad-CAM can effectively fool CNNs. This reveals an important fact that CNNs have the characteristics of huma...
PUM
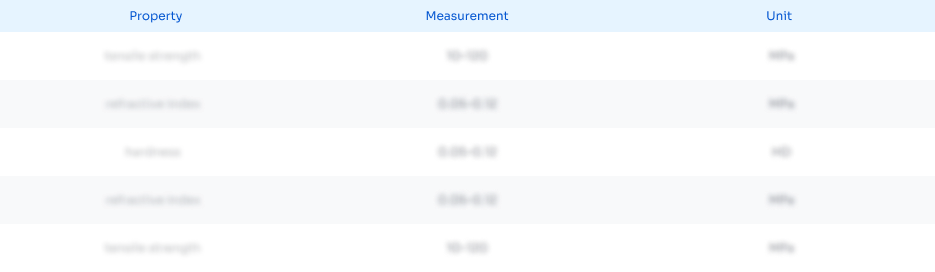
Abstract
Description
Claims
Application Information
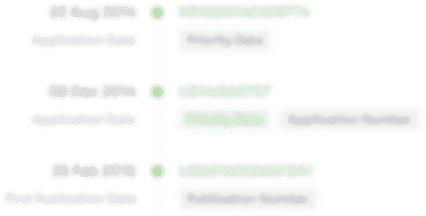
- R&D
- Intellectual Property
- Life Sciences
- Materials
- Tech Scout
- Unparalleled Data Quality
- Higher Quality Content
- 60% Fewer Hallucinations
Browse by: Latest US Patents, China's latest patents, Technical Efficacy Thesaurus, Application Domain, Technology Topic, Popular Technical Reports.
© 2025 PatSnap. All rights reserved.Legal|Privacy policy|Modern Slavery Act Transparency Statement|Sitemap|About US| Contact US: help@patsnap.com