Court electronic file oriented case information automatic extraction method
An automatic extraction and information extraction technology, applied in the field of artificial intelligence text information extraction, can solve problems such as poor normalization ability, large differences in the recognition performance of different named entities, and large impact on the training effect of language representation semantic granularity models.
- Summary
- Abstract
- Description
- Claims
- Application Information
AI Technical Summary
Problems solved by technology
Method used
Image
Examples
Embodiment Construction
[0075] The following will clearly and completely describe the technical solutions in the embodiments of the present invention with reference to the accompanying drawings in the embodiments of the present invention. Obviously, the described embodiments are only some, not all, embodiments of the present invention. All other embodiments obtained by persons of ordinary skill in the art based on the embodiments of the present invention belong to the protection scope of the present invention.
[0076] like Figure 1-4 As shown, according to the method for automatically extracting case information for court electronic files described in the embodiment of the present invention, the method includes:
[0077] Step 1: Create a case information extraction framework;
[0078] Step 2: Build and train NER models based on multi-granularity semantic legal documents.
[0079] In a specific embodiment of the present invention,
[0080] Step 1: Create a case information extraction framework; ...
PUM
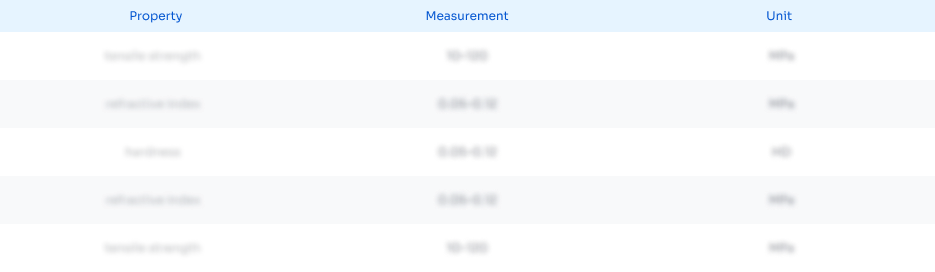
Abstract
Description
Claims
Application Information
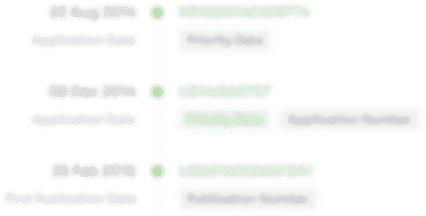
- R&D Engineer
- R&D Manager
- IP Professional
- Industry Leading Data Capabilities
- Powerful AI technology
- Patent DNA Extraction
Browse by: Latest US Patents, China's latest patents, Technical Efficacy Thesaurus, Application Domain, Technology Topic, Popular Technical Reports.
© 2024 PatSnap. All rights reserved.Legal|Privacy policy|Modern Slavery Act Transparency Statement|Sitemap|About US| Contact US: help@patsnap.com