Melanoma segmentation method based on cavity convolution and multi-scale fusion
A multi-scale fusion, melanoma technology, applied in neural learning methods, image analysis, character and pattern recognition, etc., can solve the problems of information redundancy, blurred borders of melanoma regions, different shapes, etc., to suppress information redundancy. Effects of noise, mitigation of insufficient features, and reduction of semantic gaps
- Summary
- Abstract
- Description
- Claims
- Application Information
AI Technical Summary
Problems solved by technology
Method used
Image
Examples
Embodiment Construction
[0028] The present invention will be further described below in conjunction with accompanying drawing:
[0029] The melanoma segmentation method based on hole convolution and multi-scale fusion of the present invention comprises the following steps:
[0030] Step 1) preprocessing medical images;
[0031] Divide the collected dermoscopic image data into training set, verification set and test set according to 7:1:2, and set the image pixel size to 128*128; do data augmentation on the training set images used for network training Processing, random rotation in the range of -30° to 30°, random horizontal flip and random scaling to between 0.8 and 1.2 times the original image;
[0032] Step 2) constructing a multi-scale aggregation network model with flexible receptive fields;
[0033] 2.1 Construct a channel attention hole convolution module for feature extraction;
[0034] Replace the encoding layer in U-Net with the channel attention hole convolution layer, take the dermosco...
PUM
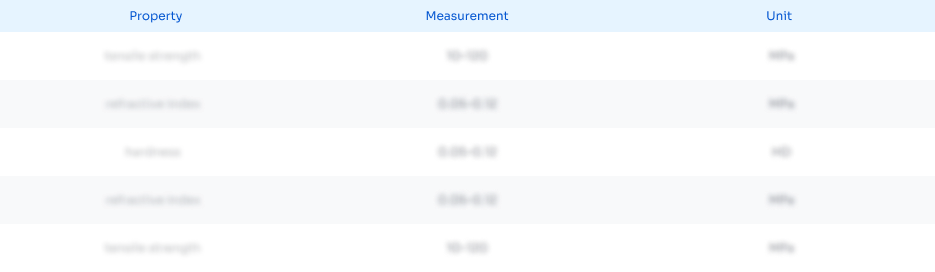
Abstract
Description
Claims
Application Information
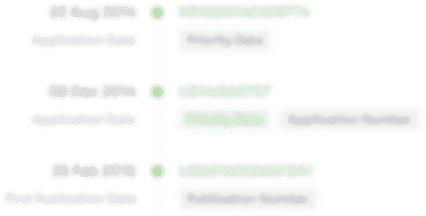
- R&D Engineer
- R&D Manager
- IP Professional
- Industry Leading Data Capabilities
- Powerful AI technology
- Patent DNA Extraction
Browse by: Latest US Patents, China's latest patents, Technical Efficacy Thesaurus, Application Domain, Technology Topic, Popular Technical Reports.
© 2024 PatSnap. All rights reserved.Legal|Privacy policy|Modern Slavery Act Transparency Statement|Sitemap|About US| Contact US: help@patsnap.com