Fatigue reliability analysis method of steel box girder based on two-stage convergence criterion
An analysis method and technology of steel box girder, applied in complex mathematical operations, design optimization/simulation, calculation, etc., can solve problems such as inefficient construction of DoE, improve reliability analysis efficiency, improve automation and intelligence, and analyze Efficiency, the effect of improving sampling efficiency
- Summary
- Abstract
- Description
- Claims
- Application Information
AI Technical Summary
Problems solved by technology
Method used
Image
Examples
Embodiment 1
[0083] Embodiment 1 of the present invention: this embodiment is a steel box girder fatigue reliability analysis method based on two-stage convergence criteria, such as figure 1 As shown, the reliability evaluation problem of extremely small failure probability events is dealt with by using the subset simulation outer framework, and the active learning method is used to select sample points in each subset to train the internal kriging model, and a two-stage Convergence criteria to control hierarchical and global estimation errors during subset simulations. Specifically include:
[0084] Step 1: Initialize the internal Kriging proxy model, use the U function to continuously increase the sample points near the failure surface for training by means of active learning, and obtain the estimation error of the failure probability;
[0085] Step 2: By defining intermediate failure events, constructing a subset to simulate the external framework, transforming the extremely small failu...
Embodiment 2
[0088] Step one specifically includes:
[0089] As a commonly used surrogate model, the kriging model can approximate the complex input-output function relationship and replace the limit state function. In kriging theory, an accurate limit state function is usually modeled as a stochastic process consisting of two parts: linear regression to the global mean and stochastic modeling of the local bias, which can be expressed as:
[0090] G(x)=f(x) T β+Z(x) (1)
[0091] where f(x) T is a set of known basis function vectors, β is the regression parameter vector, Z(x) is a stationary Gaussian random process, and its covariance function is established based on the Gaussian correlation function, which can be calculated according to the following formula:
[0092] cov(Z(x i ), Z(x j )) = σ 2 R(x i , x j ) (2)
[0093] In the formula, σ 2 is the variance of the random process, R(x i , x j ) is a Gaussian correlation function. Based on the least squares regression algorithm,...
Embodiment 3
[0113] Step two specifically includes:
[0114] The samples of the first layer of the subset simulation are directly generated by the Monte Carlo simulation, so the samples are independent of each other. However, the samples of other layers are generated by Markov chain Monte Carlo simulation, and the first samples of different Markov chains are correlated, so the samples of other layers are correlated. As a simplifying condition, the present invention assumes that the sample-based adjustment functions are independent, namely
[0115] cov(P adj (xi), P adj (x j ))=0, i≠j (11)
[0116] In order to study the influence of the randomness of the Kriging model on the estimation of the failure probability, the expectation of the adjustment function is defined as
[0117] E. adj =E[P adj (x)]=∫P adj (x)f X (x)dx (12)
[0118] Among them, f X (x) is the joint probability density function of random variable X, that is, for the first layer of subset simulation, f X (x)=q X (...
PUM
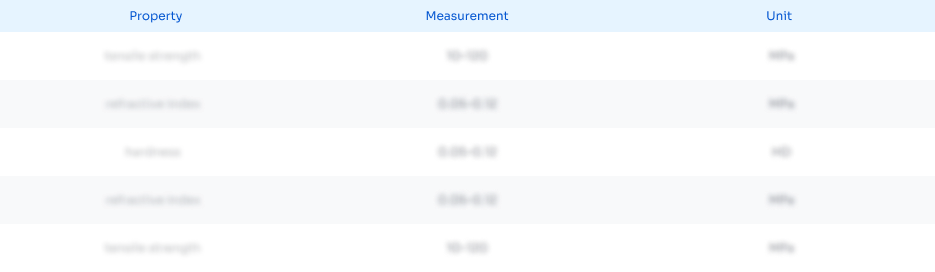
Abstract
Description
Claims
Application Information
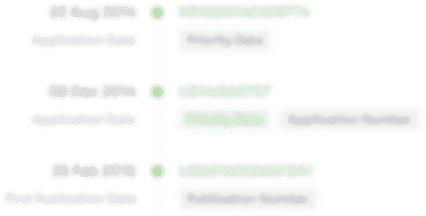
- Generate Ideas
- Intellectual Property
- Life Sciences
- Materials
- Tech Scout
- Unparalleled Data Quality
- Higher Quality Content
- 60% Fewer Hallucinations
Browse by: Latest US Patents, China's latest patents, Technical Efficacy Thesaurus, Application Domain, Technology Topic, Popular Technical Reports.
© 2025 PatSnap. All rights reserved.Legal|Privacy policy|Modern Slavery Act Transparency Statement|Sitemap|About US| Contact US: help@patsnap.com