Multichannel time series data fault diagnosis method based on convolutional neural network
A convolutional neural network, time series technology, applied in the field of multi-channel time series data fault diagnosis based on convolutional neural network, can solve problems such as occupation, Softmax classification function SVM is powerful, and the effect of fault diagnosis is limited.
- Summary
- Abstract
- Description
- Claims
- Application Information
AI Technical Summary
Problems solved by technology
Method used
Image
Examples
Embodiment Construction
[0031] In order to better understand the present invention, the present invention will be further described below in conjunction with the accompanying drawings and embodiments. It should be understood that the specific embodiments described here are only used to explain the present invention, not to limit the present invention. In addition, the technical features involved in the various embodiments of the present invention described below can be combined with each other as long as they do not constitute a conflict with each other.
[0032] Please refer to the attached figure 1 ~ attached Figure 10 , the multi-channel time series data fault diagnosis method based on the convolutional neural network provided by the preferred embodiment of the present invention, such as figure 1 , which includes the following steps:
[0033] Step (1), collecting the multi-channel one-dimensional time-series fault data of the monitoring object; arranging sensors on the monitoring object, using...
PUM
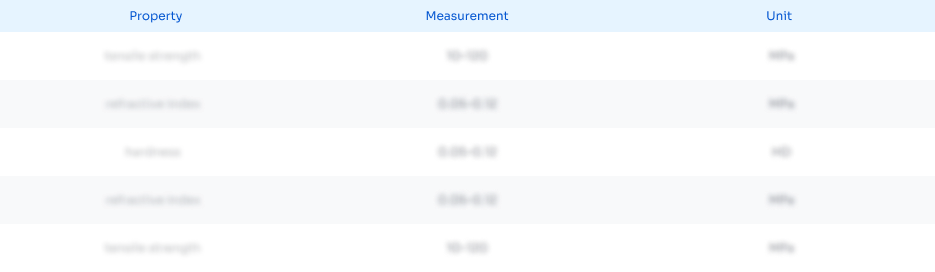
Abstract
Description
Claims
Application Information
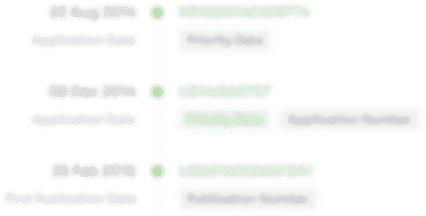
- Generate Ideas
- Intellectual Property
- Life Sciences
- Materials
- Tech Scout
- Unparalleled Data Quality
- Higher Quality Content
- 60% Fewer Hallucinations
Browse by: Latest US Patents, China's latest patents, Technical Efficacy Thesaurus, Application Domain, Technology Topic, Popular Technical Reports.
© 2025 PatSnap. All rights reserved.Legal|Privacy policy|Modern Slavery Act Transparency Statement|Sitemap|About US| Contact US: help@patsnap.com