Multi-class model training method based on gradient balance, medium and equipment
A model training, multi-category technology, applied in computational models, biological neural network models, character and pattern recognition, etc., can solve the problem that the model is difficult to learn difficult samples, reduce the model's ability to learn simple samples, etc., to improve the generalization ability. and accuracy, shortening training time, and improving accuracy
- Summary
- Abstract
- Description
- Claims
- Application Information
AI Technical Summary
Problems solved by technology
Method used
Image
Examples
Embodiment 1
[0037] Embodiments of the present invention provide a multi-category model training method based on gradient balance, which can be widely used in computer equipment, such as personal computers, cloud servers, computing clusters, or other electronic equipment that supports executable model training middle.
[0038] Such as figure 1 Shown, described training method comprises the steps:
[0039] S1: Obtain the loss function by dividing the training sample data into several batches and inputting it to the selected neural network model for training;
[0040] Wherein, the present embodiment adopts the OpenImage data set with multiple categories and large amount of data as a training sample, and the neural network model is a machine learning model based on the chain rule and stochastic gradient descent training methods, such as VGG, Inception, ResNet Convolutional neural network models.
[0041] S2: The gradient of the loss function when statistically training the model;
[0042]...
Embodiment 2
[0064] Based on the above model training method, Embodiment 2 of the present invention provides a computer-readable storage medium storing a computer program, and when the program is executed by a processor, the gradient balance-based multi-category model training method is implemented.
Embodiment 3
[0066] Based on the above model training method, Embodiment 3 of the present invention provides an electronic device, such as figure 2 As shown, the device includes a memory, a processor and a display;
[0067] The memory stores a computer program of an algorithm;
[0068] The processor is connected with the memory data, and executes the multi-category model training method based on gradient balance according to any one of claims 1-7 when calling the computer program.
[0069] The display is connected to the processor and the memory data, and displays an operation interaction interface related to the gradient balance-based multi-category model training method.
PUM
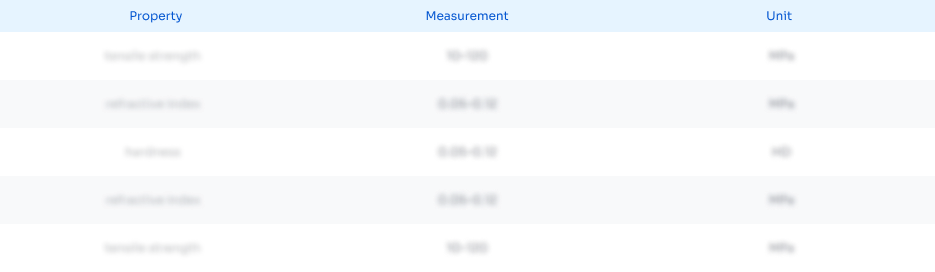
Abstract
Description
Claims
Application Information
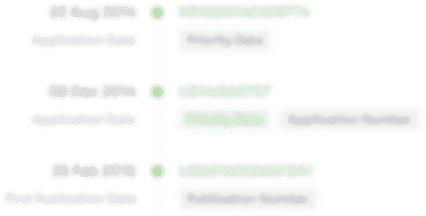
- R&D
- Intellectual Property
- Life Sciences
- Materials
- Tech Scout
- Unparalleled Data Quality
- Higher Quality Content
- 60% Fewer Hallucinations
Browse by: Latest US Patents, China's latest patents, Technical Efficacy Thesaurus, Application Domain, Technology Topic, Popular Technical Reports.
© 2025 PatSnap. All rights reserved.Legal|Privacy policy|Modern Slavery Act Transparency Statement|Sitemap|About US| Contact US: help@patsnap.com