Railway tunnel crack detection method based on improved residual network
A detection method and tunnel technology, applied in neural learning methods, biological neural network models, image data processing, etc., can solve problems such as loss of details, missed detection of small cracks, failure to find image features of railway tunnels, etc., to achieve good classification and increase The effect of accuracy
- Summary
- Abstract
- Description
- Claims
- Application Information
AI Technical Summary
Problems solved by technology
Method used
Image
Examples
Embodiment 1
[0049] Such as figure 1 As described above, step 100 is executed to collect images of railway tunnel cracks and obtain a railway tunnel crack detection data set.
[0050] Execute step 110, perform enhancement processing on the images in the railway tunnel crack detection data set and randomly divide the images into a training set and a test set. The enhancement processing includes performing at least one of gray scale processing, Gaussian filtering and image normalization on the image.
[0051] Execute step 120 to improve the residual network structure, use the images in the training set to train the improved residual network, continuously optimize the residual network structure through training, and use the test set images to test the accuracy of the residual network after the training is completed . The improved residual network structure is that the pyramid hole convolution module is integrated into the bottom of ResNet, the number of convolution kernels of each convoluti...
Embodiment 2
[0072] Aiming at the characteristics of the railway tunnel crack image, the present invention proposes a railway tunnel crack detection method based on the improved residual network (Pyramid Dilated Convolution Residual Network, PDC-ResNet), the method improves the ResNet residual network structure, and the main improvement aspects are : Combine dilated convolution blocks with different expansion rates with traditional convolutional blocks to form a pyramidal dilated convolution module; add a pyramid dilated convolution module to improve the underlying receptive field of the ResNet network and improve classification accuracy; use metrics-based The learned combination loss function is used to distinguish similar differences between different classes, reduce the missed detection rate and false detection rate of cracks, and thus better realize the detection of small cracks in complex backgrounds.
[0073] To achieve the above object, the present invention is achieved through the f...
Embodiment 3
[0107] The present invention provides a railway tunnel crack detection method based on the improved residual network. Compared with the existing technology, it has the following obvious advantages and beneficial effects:
[0108] (1) Add pyramidal hole convolution modules with different expansion rates at the bottom of ResNet to ensure that the resolution of the feature map is not reduced, and the receptive field of the convolution kernel can be expanded, which can be well used for the extraction of multi-scale features of tunnel crack images , to increase the classification accuracy.
[0109] (2) By designing a combined loss function based on metric learning, the model can increase the distance between different classes as much as possible during training to better classify small cracks in complex backgrounds.
[0110] (3) Compared with the ResNet basic network, the present invention can improve the accuracy of railway tunnel crack identification, and can effectively and time...
PUM
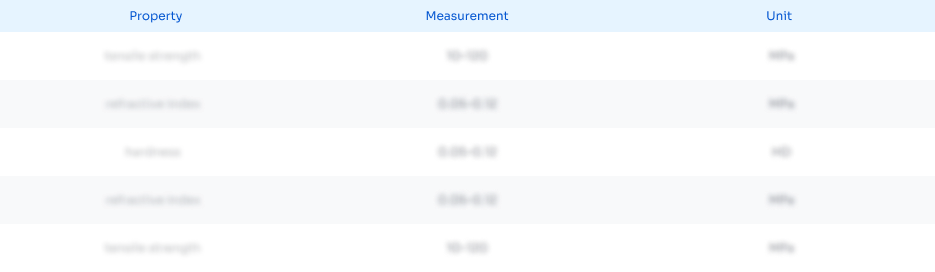
Abstract
Description
Claims
Application Information
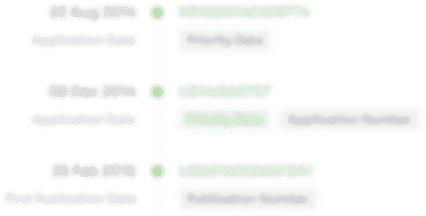
- R&D
- Intellectual Property
- Life Sciences
- Materials
- Tech Scout
- Unparalleled Data Quality
- Higher Quality Content
- 60% Fewer Hallucinations
Browse by: Latest US Patents, China's latest patents, Technical Efficacy Thesaurus, Application Domain, Technology Topic, Popular Technical Reports.
© 2025 PatSnap. All rights reserved.Legal|Privacy policy|Modern Slavery Act Transparency Statement|Sitemap|About US| Contact US: help@patsnap.com