Industrial control flow anomaly detection method and system based on convolution time sequence network
A technology of abnormal traffic and time series network, which is applied in the field of network information security, can solve problems such as loss of data information, failure to fully consider the relationship between data before and after, and achieve the effect of rapid defense measures
- Summary
- Abstract
- Description
- Claims
- Application Information
AI Technical Summary
Problems solved by technology
Method used
Image
Examples
Embodiment 1
[0052] Such as figure 1 As shown, Embodiment 1 is a method for abnormal detection of industrial control traffic based on a convolutional time series network, including:
[0053] Step 1, taking the industrial control traffic data packet to be detected as input, and splitting, merging, regularizing and grouping the data packet.
[0054] Step 2: Use the traffic data packets obtained in Step 1 as input to form a data set, use the deep learning model with decoding and encoding to learn the temporal and spatial characteristics of the data set, and predict the data corresponding to the next stage of data based on this Traffic, get the traffic forecasting model.
[0055] Step 3: Use the flow prediction model obtained in step 2 to predict the flow to be detected to obtain the prediction result, and compare the prediction result with the real flow to obtain the distance gap. Calculate the normalized score for the gap information within the group to obtain the distance gap distribution...
Embodiment 2
[0081] The second embodiment is to conduct an experiment on the industrial control data of a simulated oil refinery with S7 as the main industrial control protocol, and use the following precision to evaluate the effect of the model.
[0082] First define the following four sample sets:
[0083] 1) TP: A collection of positive samples in the data set and classified as positive by the model.
[0084] 2) FP: The set of negative samples in the data set but classified as positive by the model.
[0085] 3) TN: A set of negative samples in the data set and classified as negative by the model.
[0086] 4) FN: A collection of positive samples in the data set but classified as negative by the model.
[0087] Based on the above four sample sets, the present invention uses four evaluation indexes commonly used in the field of intrusion detection to evaluate the detection performance of the intrusion detection model in the present invention.
[0088] Model detection performance evaluat...
PUM
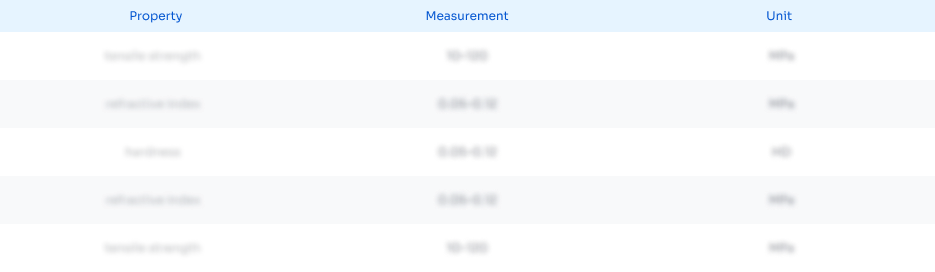
Abstract
Description
Claims
Application Information
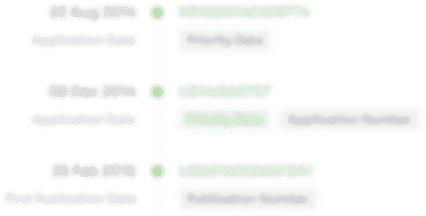
- R&D
- Intellectual Property
- Life Sciences
- Materials
- Tech Scout
- Unparalleled Data Quality
- Higher Quality Content
- 60% Fewer Hallucinations
Browse by: Latest US Patents, China's latest patents, Technical Efficacy Thesaurus, Application Domain, Technology Topic, Popular Technical Reports.
© 2025 PatSnap. All rights reserved.Legal|Privacy policy|Modern Slavery Act Transparency Statement|Sitemap|About US| Contact US: help@patsnap.com