Multi-modal medical image fusion based on multi-CNN combination and fuzzy neural network
A fuzzy neural network and medical image technology, applied in the field of medical image fusion, can solve the problems of information loss, difficult clinical diagnosis of images, and single convolution kernel setting.
- Summary
- Abstract
- Description
- Claims
- Application Information
AI Technical Summary
Problems solved by technology
Method used
Image
Examples
Embodiment Construction
[0057] Such as figure 1 Shown, the multimodal medical image fusion based on multi-CNN combination and fuzzy neural network of the present invention, comprises the following steps:
[0058] Step 1, after filtering the CT and MR images of the training data set through a filter bank composed of 16 two-dimensional Gabor filters with different scales and directions, the formula is:
[0059]
[0060] In formula (1), U represents the direction of the filter bank, which is selected as 0°, 90°, 180° and 270°; V represents the scale of the filter bank, which is set to 4, 8, 16 and 32; z=( x, y) represents the position of the pixel, k v =k max / f v , φ u = πu / 8,k max is the maximum frequency and f is the spacing factor between filters in the frequency domain. According to formula (1), it can be seen that the filter can be set with different U directions and V scales, showing different Gabor representations of medical images.
[0061] Gabor representations of CT and MR at u a...
PUM
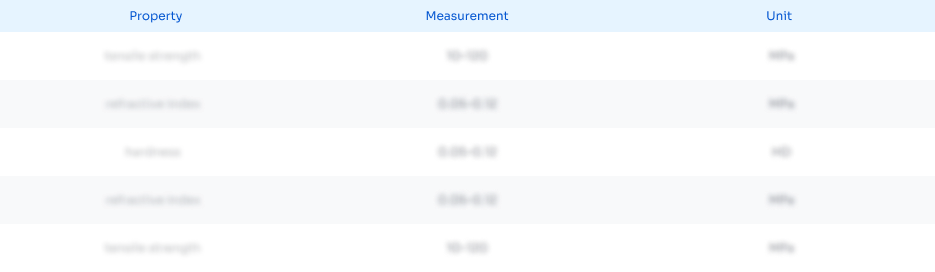
Abstract
Description
Claims
Application Information
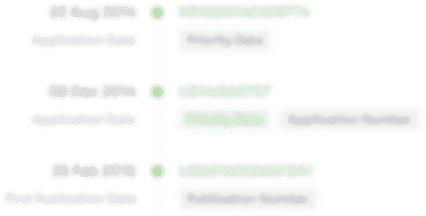
- R&D Engineer
- R&D Manager
- IP Professional
- Industry Leading Data Capabilities
- Powerful AI technology
- Patent DNA Extraction
Browse by: Latest US Patents, China's latest patents, Technical Efficacy Thesaurus, Application Domain, Technology Topic, Popular Technical Reports.
© 2024 PatSnap. All rights reserved.Legal|Privacy policy|Modern Slavery Act Transparency Statement|Sitemap|About US| Contact US: help@patsnap.com