Medical image segmentation or classification method based on small sample domain self-adaption
A domain-adaptive and medical imaging technology, which is applied in the field of medical image segmentation or classification based on small-sample domain-adaptive, can solve the problems of high labeling costs for doctors, biased diagnostic results, and inability to guarantee diagnostic accuracy, etc.
- Summary
- Abstract
- Description
- Claims
- Application Information
AI Technical Summary
Problems solved by technology
Method used
Image
Examples
Embodiment Construction
[0031] The present invention will be further described below in conjunction with the accompanying drawings and specific embodiments.
[0032] The present invention builds a small-sample domain adaptive model based on a deep convolutional neural network. The input of the small-sample domain adaptive model includes a labeled source domain training data set and a target domain data set, wherein the target domain data set includes training data with known labels. The training set with unknown set and label, the output layer of the small-sample domain adaptive model is the Softmax layer. The small-sample domain adaptive model is used as a black box, without artificially specifying the use of certain lesion features for classification, and the computer can learn the process of classification or segmentation by itself. At the same time, the input of the small-sample domain adaptive model is the entire image, not the image. A certain feature can reduce the loss of information. When t...
PUM
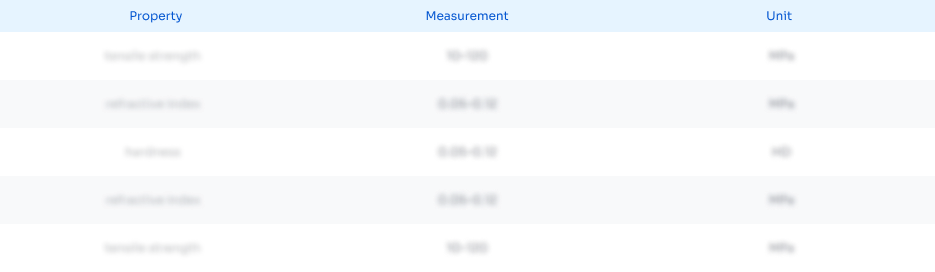
Abstract
Description
Claims
Application Information
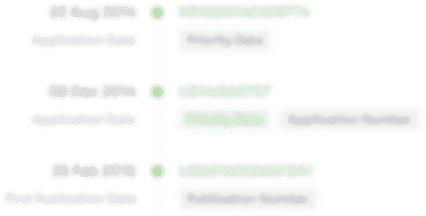
- R&D
- Intellectual Property
- Life Sciences
- Materials
- Tech Scout
- Unparalleled Data Quality
- Higher Quality Content
- 60% Fewer Hallucinations
Browse by: Latest US Patents, China's latest patents, Technical Efficacy Thesaurus, Application Domain, Technology Topic, Popular Technical Reports.
© 2025 PatSnap. All rights reserved.Legal|Privacy policy|Modern Slavery Act Transparency Statement|Sitemap|About US| Contact US: help@patsnap.com