Lightweight neural network hardware accelerator based on depth separable convolution
A technology of convolutional neural network and hardware accelerator, applied in the direction of biological neural network model, neural architecture, neural learning method, etc., can solve the problems of low utilization rate of systolic array, cost and energy loss, etc., and achieve the reduction of high power consumption off-chip The effect of accessing memory, saving resources, and improving processing performance
- Summary
- Abstract
- Description
- Claims
- Application Information
AI Technical Summary
Problems solved by technology
Method used
Image
Examples
Embodiment Construction
[0028] The present invention will be further described below in conjunction with accompanying drawing.
[0029] In this example, if image 3 and Figure 4 As shown, a lightweight neural network hardware accelerator based on depthwise separable convolution, including a parallel array of A-way K×K-channel convolution processing units, a parallel array of A-way 1×1 point convolution processing units, and a buffer for On-chip memory for convolutional neural networks and input-output feature maps. The convolutional neural network is a lightweight neural network obtained by compressing the neural network MobileNet using a quantization-aware training method.
[0030] Such as Figure 4 As shown, the parallel array of A-way K×K channel convolution processing units and the parallel array of multiple 1×1 point convolution processing units are deployed in a pixel-level pipeline.
[0031] Such as Figure 5 As shown, each K×K channel convolution processing unit in the A-way K×K channel...
PUM
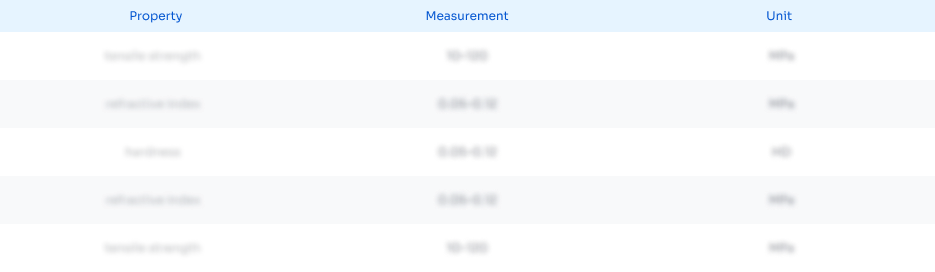
Abstract
Description
Claims
Application Information
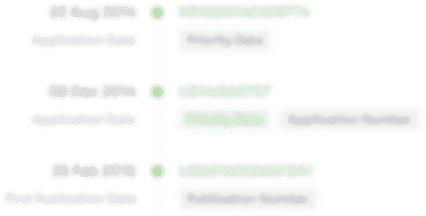
- R&D Engineer
- R&D Manager
- IP Professional
- Industry Leading Data Capabilities
- Powerful AI technology
- Patent DNA Extraction
Browse by: Latest US Patents, China's latest patents, Technical Efficacy Thesaurus, Application Domain, Technology Topic, Popular Technical Reports.
© 2024 PatSnap. All rights reserved.Legal|Privacy policy|Modern Slavery Act Transparency Statement|Sitemap|About US| Contact US: help@patsnap.com