RGB-D image saliency target detection method based on cross-modal feature fusion
A technology of RGB-D and RGB images, which is applied in the field of saliency target detection of RGB-D images based on cross-modal feature fusion, which can solve problems such as insufficient use of Depth cues.
- Summary
- Abstract
- Description
- Claims
- Application Information
AI Technical Summary
Problems solved by technology
Method used
Image
Examples
Embodiment 1
[0037] like Figure 1-4 As shown, a RGB-D image salient target detection method based on cross-modal feature fusion:
[0038] Step 1. Based on the U-Net network of the cross-layer connection method, input RGB and Depth into the ResNet-50 backbone network to extract image features, among which the five-stage features extracted from the RGB image are R1, R2, R3, and R4. , R5; the five-stage features extracted from the Depth image are D1, D2, D3, D4, and D5; the U-Net network is carried out on the U-shaped architecture of the codec, where the encoder process is divided into two paths , for ResNet-50 processing the RGB image path and ResNet-50 processing the RGB path, respectively.
[0039] Step 2. Input the top-level features R5 and D5 under the two modalities into the cross-modal channel refinement module to obtain the cross-modal feature RD; guide D1-D5 and RD in the Depth mode through the cross-modal guidance module to guide RGB Feature extraction; considering the strong com...
Embodiment 2
[0076] Step 1. Based on the U-Net network of the cross-layer connection method, input RGB and Depth into the ResNet-50 backbone network to extract image features, among which the five-stage features extracted from the RGB image are R1, R2, R3, and R4. , R5; Depth image extracts the features of the five stages are D1, D2, D3, D4, D5;
[0077] Step 2. Input the top-level features R5 and D5 under the two modalities into the cross-modal channel refinement module to obtain the cross-modal feature RD; guide D1-D5 and RD in the Depth mode through the cross-modal guidance module to guide RGB Perform feature extraction;
[0078] Step 3. Use R1~R5 and RD in the RGB mode to further retain the foreground salient information of the image through the residual adaptive selection module, and discard the disturbing background information to obtain U1, U2, U3, U4, and U5; through five The cross-entropy loss function performs supervised learning on U1~U5 respectively, and guides the network to ...
PUM
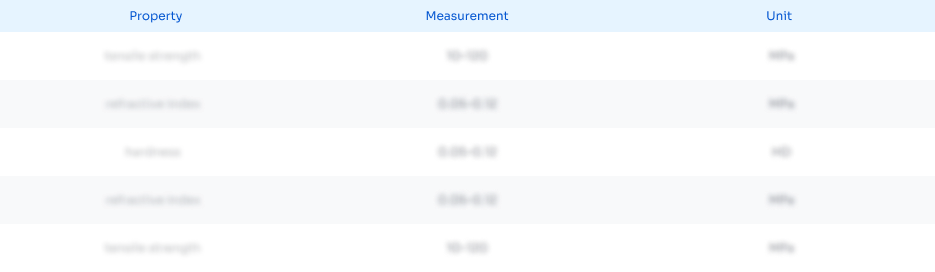
Abstract
Description
Claims
Application Information
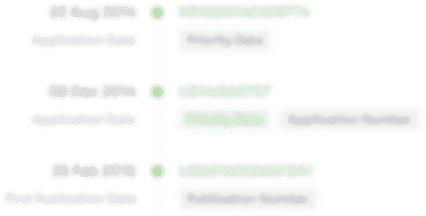
- R&D Engineer
- R&D Manager
- IP Professional
- Industry Leading Data Capabilities
- Powerful AI technology
- Patent DNA Extraction
Browse by: Latest US Patents, China's latest patents, Technical Efficacy Thesaurus, Application Domain, Technology Topic, Popular Technical Reports.
© 2024 PatSnap. All rights reserved.Legal|Privacy policy|Modern Slavery Act Transparency Statement|Sitemap|About US| Contact US: help@patsnap.com