Recommendation system based on non-sampling collaborative knowledge graph network
A recommendation system and knowledge graph technology, applied in the field of recommendation, can solve the problems of errors, large memory and time costs, and will not bring profitability, and achieve the effect of avoiding errors and achieving good speed.
- Summary
- Abstract
- Description
- Claims
- Application Information
AI Technical Summary
Problems solved by technology
Method used
Image
Examples
example
[0047] Calculate the attention parameter π(h, r, t) using the nonlinear activation function tanh: π(h, r, t) = (W r e t ) T tanh (W r e h +e t );
[0048] It can be seen that the attention score is determined by e in the relational space h and e t determined by the distance.
[0049] Then through the softmax activation function, the coefficients of the entire triplet are normalized: Among them, N h is the triplet set with entity h as the head entity; r', t' are other relations and tail entities in the triplet set with entity h as the head entity.
[0050] In this application, in order not to destroy the efficient precomputation during the graph aggregation operation, the solution of the present invention only needs to predetermine the attention parameters through a small subset of the training graph, and then proceed to the next step.
[0051] The information propagation component is configured to calculate an initial propagation matrix B according to the attention ...
Embodiment
[0072] The present invention provides a recommendation system based on a non-sampling collaborative knowledge graph network, such as figure 2 As shown, this embodiment also provides a comparison between the technical solution of the present invention and the prior art, and evaluates the performance of the model for three real data sets of music, books and movies. For the convenience of brief description, a non-sampling collaborative knowledge graph network (Non-Sampling Collaborative Knowledge Graph Network) proposed by the present invention is referred to as NCKN for short.
[0073] In this example, the following three real data sets are used to evaluate the model performance: Last.FM (Music), Book-Crossing (Book), MovieLens-20M (Movie), as described in Table 1, the relevant statistics are given . All three datasets are publicly accessible and vary in size and sparsity.
[0074] (1) Last.FM: User listening behavior and project knowledge provided by the Last.FM online music...
PUM
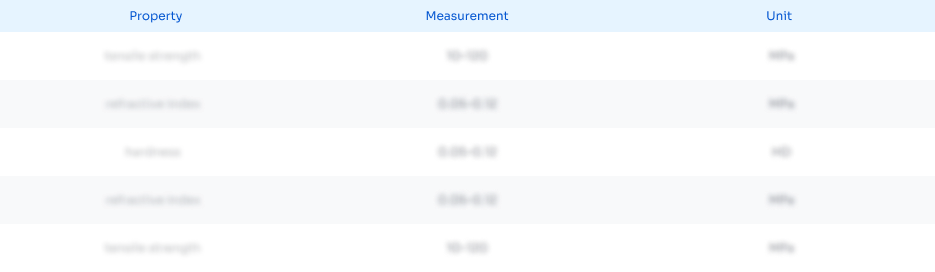
Abstract
Description
Claims
Application Information
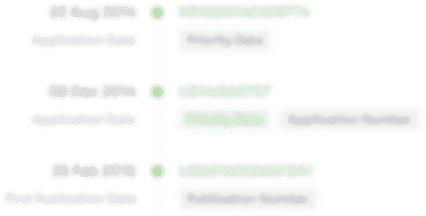
- R&D Engineer
- R&D Manager
- IP Professional
- Industry Leading Data Capabilities
- Powerful AI technology
- Patent DNA Extraction
Browse by: Latest US Patents, China's latest patents, Technical Efficacy Thesaurus, Application Domain, Technology Topic, Popular Technical Reports.
© 2024 PatSnap. All rights reserved.Legal|Privacy policy|Modern Slavery Act Transparency Statement|Sitemap|About US| Contact US: help@patsnap.com