Mesoscale vortex trajectory stationary sequence extraction and recurrent neural network prediction method
A technology of cyclic neural network and prediction method, which is applied in the field of mesoscale vortex trajectory smooth sequence extraction and cyclic neural network prediction, which can solve the problems of complex multi-step prediction, mesoscale vortex without periodicity, moving speed and self-transformation are not fixed, etc.
- Summary
- Abstract
- Description
- Claims
- Application Information
AI Technical Summary
Problems solved by technology
Method used
Image
Examples
Embodiment 1
[0108] Regarding data collection: the present invention collects the mesoscale vortex trajectory data retrieved from the AVISO satellite altimeter (Chelton et al., 2011), the mesoscale vortex trajectory attribute description data including amplitude, rotation speed, radius and latitude and longitude, and the data from ETOPO1 The oceanic bathymetric data, involving the topographic bathymetric data corresponding to the longitude and latitude positions of the mesoscale eddy track. Among them, the mesoscale vortex amplitude represents the difference between the maximum Sea Surface Height (SSH) and the SSH average value in the mesoscale vortex, and the rotation velocity represents the maximum average geostrophic velocity around all closed contours of the SSH in the mesoscale vortex , and the radius represents the radius of the circle inside the closed contour of the SSH when the mesoscale vortex reaches the maximum mean geostrophic velocity.
[0109] Example 1: The trajectory of th...
PUM
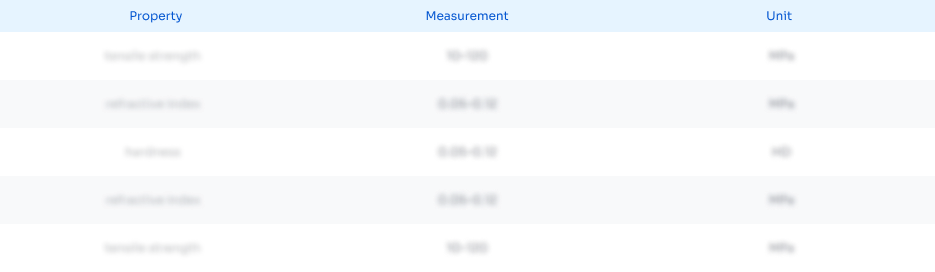
Abstract
Description
Claims
Application Information
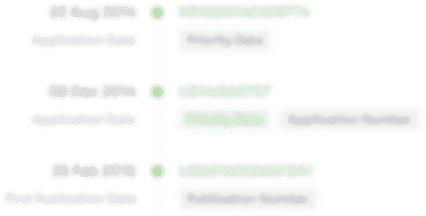
- R&D
- Intellectual Property
- Life Sciences
- Materials
- Tech Scout
- Unparalleled Data Quality
- Higher Quality Content
- 60% Fewer Hallucinations
Browse by: Latest US Patents, China's latest patents, Technical Efficacy Thesaurus, Application Domain, Technology Topic, Popular Technical Reports.
© 2025 PatSnap. All rights reserved.Legal|Privacy policy|Modern Slavery Act Transparency Statement|Sitemap|About US| Contact US: help@patsnap.com