A marker for predicting the prognosis and suitability of immunotherapy in gastric cancer and its application
An immunotherapy and marker technology, applied in the field of biomedicine, can solve problems such as different prognosis
- Summary
- Abstract
- Description
- Claims
- Application Information
AI Technical Summary
Problems solved by technology
Method used
Image
Examples
Embodiment 1
[0062] Example 1 Downloading of gastric cancer related gene data
[0063] from the Gene Expression Comprehensive Database ( http: / / www.ncbi.nlm.nih.gov / geo / ) Download the raw data and clinical data of gastric cancer transcriptome chip GSE26901 (n=109), GSE15460 (n=248), GSE62254 (n=300), GSE15459 (n=192), GSE84437 (n=433). Download gastric cancer transcriptome FPKM sequencing data and clinical data from The Cancer Genome Atlas (TCGA).
[0064] Transcriptome FPKM sequencing data were processed using the affy software package and the input R software package, and differential gene expression analysis was performed using the limma software package. Assessment of stromal and immune cell infiltration in the tumor immune microenvironment using the ESTIMATE (Estimation of Stromal and Immune Cells in Malignant Tissue Using Expression Data) algorithm. The GSE62254 and GSE15460 datasets were combined using the sva R package to remove batch effects and a total of 548 samples were obta...
Embodiment 2
[0065] Example 2 Gastric cancer related gene data processing
[0066] 2.1 Construction of weighted gene co-expression network (WGCNA)
[0067] The gene expression data matrix of GSE26901 was constructed using the WGCNA R software package, and the genes with the variance in the top 25% were selected as the input data set for the subsequent WGCNA. Then use hierarchical clustering to remove abnormal samples, then build a scale-free network, and calculate the degree of dissimilarity of genes. According to the degree of dissimilarity, genes with similar expression profiles are modularized, and the correlation between the modules and the clinical phenotype of gastric cancer is calculated.
[0068] 2.2 Identify core modules
[0069] Input the genes in the module into the STRING (Search Tool for Retrieving Interacting Genes / Proteins) website for PPI (Protein-Protein Interaction) analysis, get a PPI score, then input the PPI score into Cytoscape for analysis with the MCODE plugin, and...
Embodiment 3
[0076] Example 3 Construction of prognostic risk model
[0077] 3.1WGCNA identified modules related to tumor progression and further screened core genes.
[0078] To identify genes associated with gastric cancer progression, a co-expression network was constructed in GSE26901 using WGCNA. After removing 5 outlier gastric cancer patient samples, the remaining 104 gastric cancer patient samples were used to construct an adjacency matrix graph ( figure 1 ). β = 9 is chosen as the soft threshold to construct the scale-free network graph ( figure 2 ), and finally constructed 10 gene co-expression modules ( image 3 ). By calculating the correlation between the gene co-expression module and clinical characteristics, it was found that the midnight-blue module had the strongest correlation with AJCC-stage ( Figure 4 ). In order to obtain the core genes, we continued to analyze the PPI network in the midnight-blue module on the STRING website, imported the results into Cytosca...
PUM
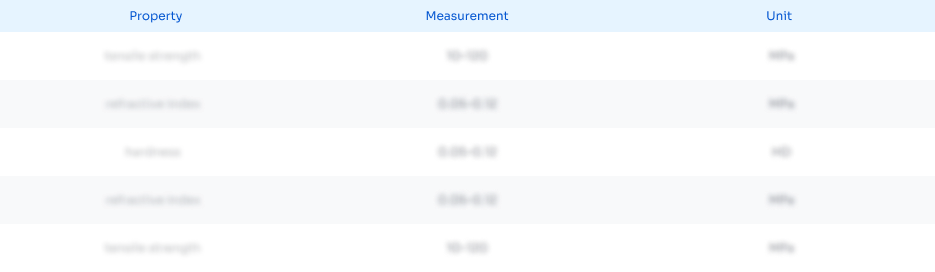
Abstract
Description
Claims
Application Information
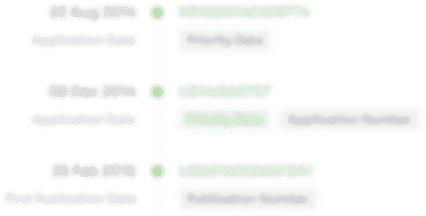
- R&D Engineer
- R&D Manager
- IP Professional
- Industry Leading Data Capabilities
- Powerful AI technology
- Patent DNA Extraction
Browse by: Latest US Patents, China's latest patents, Technical Efficacy Thesaurus, Application Domain, Technology Topic.
© 2024 PatSnap. All rights reserved.Legal|Privacy policy|Modern Slavery Act Transparency Statement|Sitemap