Multi-task learning method based on information entropy dynamic weighting
A multi-task learning and information entropy technology, which is applied in the field of multi-task learning adaptive balance based on information entropy dynamic weighting, can solve problems such as dependence on human experience, lack of adaptability, and subtasks cannot work normally, so as to improve task performance , the effect of strong applicability of the algorithm
- Summary
- Abstract
- Description
- Claims
- Application Information
AI Technical Summary
Problems solved by technology
Method used
Image
Examples
Embodiment Construction
[0061] The specific implementation method of the present invention will be further described in detail below in conjunction with the accompanying drawings and taking a multi-task learning network that jointly realizes semantic segmentation, depth estimation and edge detection in computer vision as an example.
[0062] The present invention proposes a multi-task learning self-adaptive balance method based on information entropy dynamic weighting, which adopts phased training, first uses the multi-task loss function with fixed weight for pre-training, and then uses the adaptive multi-task loss function with dynamic weighting Do dynamic training. In the process of model training, the information entropy algorithm can effectively evaluate the prediction results of each task, and adjust the relative weight of the task through the dynamic weighting strategy, so that the multi-task prediction model pays more attention to and improves the tasks with relatively poor performance, thereby...
PUM
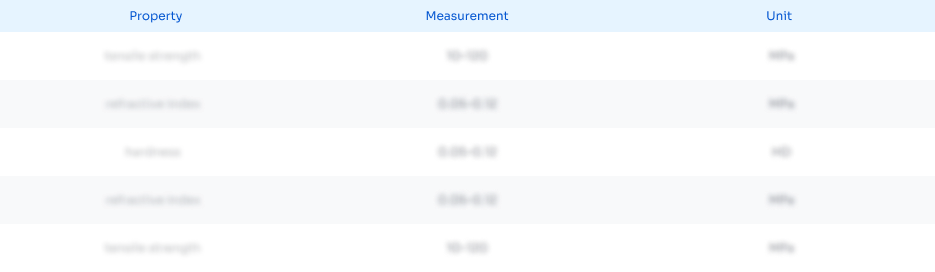
Abstract
Description
Claims
Application Information
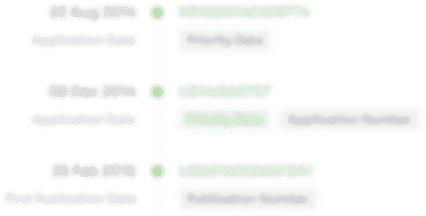
- R&D Engineer
- R&D Manager
- IP Professional
- Industry Leading Data Capabilities
- Powerful AI technology
- Patent DNA Extraction
Browse by: Latest US Patents, China's latest patents, Technical Efficacy Thesaurus, Application Domain, Technology Topic, Popular Technical Reports.
© 2024 PatSnap. All rights reserved.Legal|Privacy policy|Modern Slavery Act Transparency Statement|Sitemap|About US| Contact US: help@patsnap.com