Wind power ultra-short-term rolling prediction method based on WT-TCN
A technology for wind power and rolling forecasting, applied in forecasting, neural learning methods, electrical digital data processing, etc. Effects of stability issues
- Summary
- Abstract
- Description
- Claims
- Application Information
AI Technical Summary
Problems solved by technology
Method used
Image
Examples
Embodiment
[0055] Embodiment: According to the measured data of a certain wind farm.
[0056] A method for ultra-short-term rolling prediction of wind power based on WT-TCN, characterized in that it comprises the following steps:
[0057] 1) Decompose the output power of wind turbines in the wind farm using formula (1) using different wavelet scales to obtain low-frequency signals and high-frequency signals, and use formula (2) to conduct correlation analysis results for low-frequency signals and high-frequency signals. Figure 4 , attached Figure 5 As shown, the wavelet scale is selected according to the maximum autocorrelation coefficient, and finally the haar wavelet scale is selected:
[0058]
[0059]
[0060] In the formula: ACF is the autocorrelation coefficient; x i is the i-th sample point of the sequence; n is the total number of items; u is the mean value of the time series; b 0 and c 0 Respectively, scale factor and displacement factor; ψ is the mother wavelet func...
PUM
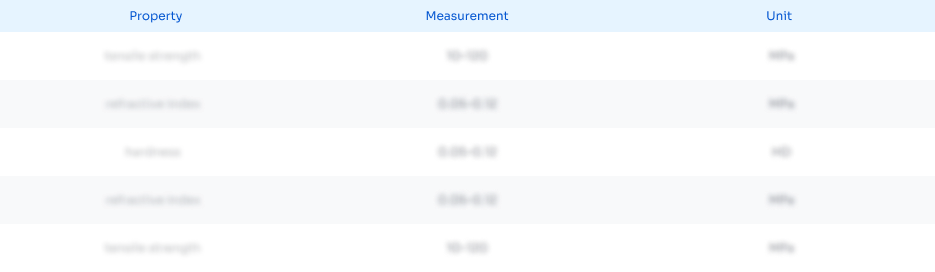
Abstract
Description
Claims
Application Information
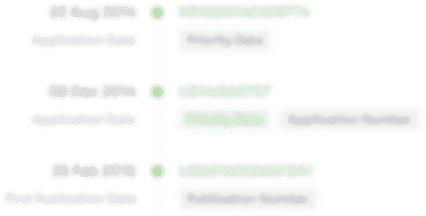
- R&D Engineer
- R&D Manager
- IP Professional
- Industry Leading Data Capabilities
- Powerful AI technology
- Patent DNA Extraction
Browse by: Latest US Patents, China's latest patents, Technical Efficacy Thesaurus, Application Domain, Technology Topic, Popular Technical Reports.
© 2024 PatSnap. All rights reserved.Legal|Privacy policy|Modern Slavery Act Transparency Statement|Sitemap|About US| Contact US: help@patsnap.com