Infrared light and visible light image fusion method based on residual dense network and gradient loss
A dense network, image fusion technology, applied in the field of image processing, which can solve problems such as reduced training speed, low quality of fusion results, and blurred edges.
- Summary
- Abstract
- Description
- Claims
- Application Information
AI Technical Summary
Problems solved by technology
Method used
Image
Examples
Embodiment 1
[0047] Such as figure 1 As shown, this embodiment provides an infrared and visible light image fusion method based on residual dense network and gradient loss, and the specific steps are as follows:
[0048] Step 1: Put the infrared light image and visible light image into the pre-trained VGG-16 network for parameter extraction, and standardize and normalize the extracted feature map values to obtain weight blocks;
[0049] Specifically, when the source image is input to the network, the source image is also passed to a specific extractor for extracting feature maps and calculating feature values for the purpose of generating parameters that need to be used in the training process. It is set that each source image extracts 5 feature maps before the 5 maximum pooling layers of the VGG-16 network. After extraction, the information contained in the feature map starts to be calculated, and their gradients are used in the calculation to work well with the gradient loss in the ...
PUM
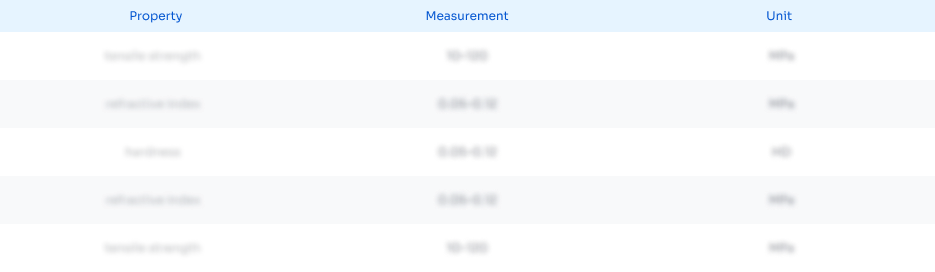
Abstract
Description
Claims
Application Information
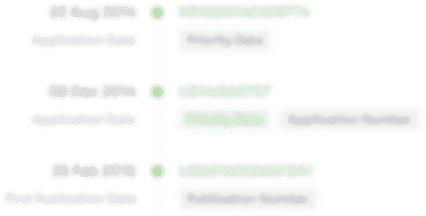
- R&D
- Intellectual Property
- Life Sciences
- Materials
- Tech Scout
- Unparalleled Data Quality
- Higher Quality Content
- 60% Fewer Hallucinations
Browse by: Latest US Patents, China's latest patents, Technical Efficacy Thesaurus, Application Domain, Technology Topic, Popular Technical Reports.
© 2025 PatSnap. All rights reserved.Legal|Privacy policy|Modern Slavery Act Transparency Statement|Sitemap|About US| Contact US: help@patsnap.com