Heterogeneous model aggregation method and system based on federated learning
A technology of heterogeneous models and aggregation methods, which is applied in neural learning methods, biological neural network models, neural architectures, etc., can solve the problem of poor fairness, does not consider the difference in client model performance, and does not better solve client data anomalies. quality and other issues, to achieve the effect of high quality and reduced communication volume
- Summary
- Abstract
- Description
- Claims
- Application Information
AI Technical Summary
Problems solved by technology
Method used
Image
Examples
Embodiment 1
[0052] Such as figure 1 As shown, the heterogeneous model aggregation method based on federated learning in this embodiment uses the Mnist data set, including the following steps:
[0053] S1. Set up local data set D on each client i And initialize a neural network model M i , where i is the serial number of the client, initialize a neural network model M on the server side; where, the neural network model M of each client i The model structure and model parameters are allowed to be different, and each client's local data set D i The distribution of data is not the same.
[0054] In this embodiment, the client is a computer with a certain computing power. For the client numbered i, the local data set D i The neural network model M initialized for a part of the data in the Mnist dataset i The structure is shown in Table 1:
[0055] Table 1
[0056]
[0057] The server is a data center with strong performance and large communication volume. The model is M, and its stru...
Embodiment 2
[0101] Based on the same inventive concept as Embodiment 1, this embodiment also proposes a heterogeneous model aggregation system based on federated learning, including:
[0102] The enhanced iteration module is used to ensure that the data categories owned by the client model in the batch iteration process are complete and the distribution of each category is even, so that the client model can continuously correct the direction of gradient descent during the training process, making the gradient towards The direction of the optimal solution is descending.
[0103] The knowledge distillation module is used to solve the reliability problem of the client model under heterogeneous conditions. In each round, each client sends prediction scores to the server, and the server uses JS functions to calculate the weight of each client model prediction score, and then calculates the global prediction score by weighted average. The server-side model performs knowledge distillation throu...
PUM
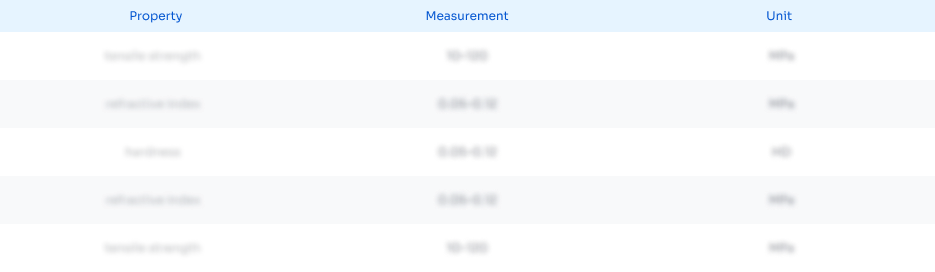
Abstract
Description
Claims
Application Information
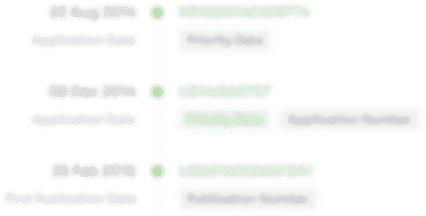
- R&D
- Intellectual Property
- Life Sciences
- Materials
- Tech Scout
- Unparalleled Data Quality
- Higher Quality Content
- 60% Fewer Hallucinations
Browse by: Latest US Patents, China's latest patents, Technical Efficacy Thesaurus, Application Domain, Technology Topic, Popular Technical Reports.
© 2025 PatSnap. All rights reserved.Legal|Privacy policy|Modern Slavery Act Transparency Statement|Sitemap|About US| Contact US: help@patsnap.com