piRNA-disease incidence relation prediction method based on convolution denoising autoencoder
A technology of association relationship and prediction method, applied in the field of deep learning and bioinformatics, can solve the problems of feature denoising and deep hidden feature extraction, time-consuming, labor-intensive, etc., to improve prediction accuracy and save time Effect
- Summary
- Abstract
- Description
- Claims
- Application Information
AI Technical Summary
Problems solved by technology
Method used
Image
Examples
Embodiment 1
[0073] refer to figure 1 As shown, this embodiment discloses a piRNA-disease correlation prediction method based on convolutional denoising autoencoder, comprising the following steps:
[0074] Step S1, selection and establishment of data sets: based on the piRDisease v1.0 database, the known association data between Piwi protein-interacting RNAs and diseases were obtained; based on the piRBase v2.0 database, the ID and sequence information of Piwi protein-interacting RNAs were obtained; based on American Medical Subject Headings (MeSH) database to obtain disease semantic information;
[0075] Step S2, generation of sequence similarity features of Piwi protein-interacting RNA: based on the nucleotide sequence information of Piwi protein-interacting RNA, that is, four nucleotide sequence information of adenine, cytosine, uracil and guanine, using the The sequence-derived feature extraction method of overlapping moving windows calculates the sequence features of each Piwi prote...
Embodiment 2
[0131] In order to better illustrate the effect of the prediction method of the present invention, this prediction method is compared with the model (contrast model) that does not use the convolution denoising auto-encoding neural network for deep feature extraction, and table 1 lists the present embodiment And compare the results generated by the model on the benchmark dataset using 5-fold cross-validation:
[0132] Table 1 Comparison of the results of the present invention and the comparison model based on the benchmark data set under the five-fold cross-validation
[0133]
[0134] figure 2 and image 3 The ROC curves generated by the present invention and the comparison model are respectively shown; it can be seen from the comparison that this embodiment has achieved higher scores on various evaluation indicators, and the results are higher than those without convolution denoising automatic encoding neural network. The comparison model of network for deep feature ext...
Embodiment 3
[0136] In order to further compare the performance of the method of the present invention, the method of the present invention is compared with two latest calculation methods, Figure 4 It shows the histogram of the AUC comparison between the two latest calculation methods and the present invention under each fold of data based on the same benchmark data set under the five-fold cross-validation; the size of the AUC value is more representative of the predictive performance of the method.
[0137] It can be seen from the comparison that the present invention has a higher AUC value than the latest calculation model, and its overall performance is better than other models.
PUM
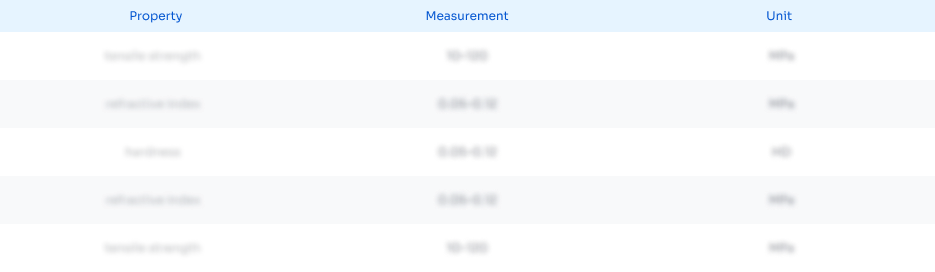
Abstract
Description
Claims
Application Information
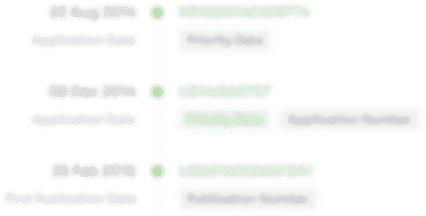
- R&D
- Intellectual Property
- Life Sciences
- Materials
- Tech Scout
- Unparalleled Data Quality
- Higher Quality Content
- 60% Fewer Hallucinations
Browse by: Latest US Patents, China's latest patents, Technical Efficacy Thesaurus, Application Domain, Technology Topic, Popular Technical Reports.
© 2025 PatSnap. All rights reserved.Legal|Privacy policy|Modern Slavery Act Transparency Statement|Sitemap|About US| Contact US: help@patsnap.com