Key electric energy equipment fault diagnosis method based on deep learning
A technology for equipment failure and diagnosis methods, applied in neural learning methods, biological neural network models, instruments, etc., can solve problems such as high management costs, complex grid structure, large amount of information and data, etc. Avoid noise interference, avoid the effect of modal aliasing
- Summary
- Abstract
- Description
- Claims
- Application Information
AI Technical Summary
Problems solved by technology
Method used
Image
Examples
Embodiment Construction
[0032] The following will clearly and completely describe the technical solutions in the embodiments of the present invention with reference to the accompanying drawings in the embodiments of the present invention. Obviously, the described embodiments are only some, not all, embodiments of the present invention. Based on the embodiments of the present invention, all other embodiments obtained by persons of ordinary skill in the art without making creative efforts belong to the protection scope of the present invention.
[0033] see Figure 1-4 , a method for diagnosing faults of key electric energy equipment based on deep learning, the method for diagnosing faults for key electric energy equipment includes the following steps:
[0034] (1) The sensors on key electric energy equipment collect data in real time.
[0035] (2) The collected data is sent to the cloud computing platform through the data transmission module, and the cloud computing technology is used to decompose th...
PUM
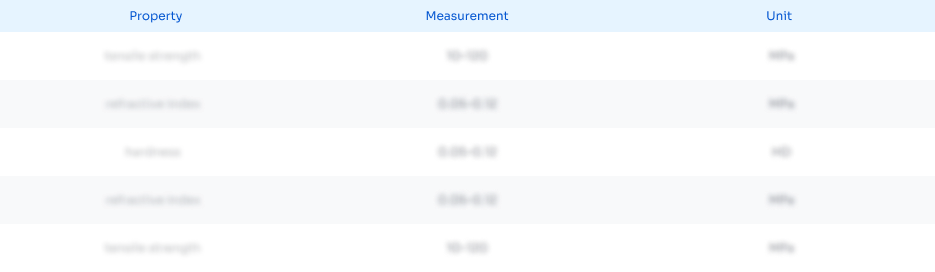
Abstract
Description
Claims
Application Information
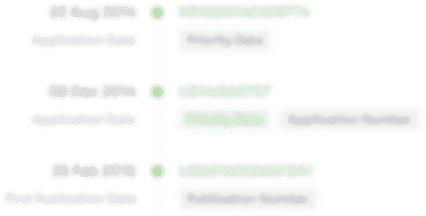
- R&D
- Intellectual Property
- Life Sciences
- Materials
- Tech Scout
- Unparalleled Data Quality
- Higher Quality Content
- 60% Fewer Hallucinations
Browse by: Latest US Patents, China's latest patents, Technical Efficacy Thesaurus, Application Domain, Technology Topic, Popular Technical Reports.
© 2025 PatSnap. All rights reserved.Legal|Privacy policy|Modern Slavery Act Transparency Statement|Sitemap|About US| Contact US: help@patsnap.com