Small sample fault diagnosis method based on task sorting meta learning
A fault diagnosis and meta-learning technology, applied in machine learning, pattern recognition in signals, instruments, etc., can solve problems such as interference and unrecognizable fault information, and achieve the effect of stable performance
- Summary
- Abstract
- Description
- Claims
- Application Information
AI Technical Summary
Problems solved by technology
Method used
Image
Examples
Embodiment Construction
[0036] In order to make the purpose, technical solutions and advantages of the present invention clearer, the present invention will be discussed in detail below in conjunction with the accompanying drawings and examples. The following examples are only descriptive, not restrictive, and cannot limit the protection scope of the present invention with this .
[0037] The present invention proposes a small-sample intelligent fault diagnosis method for an industrial system based on a Task-sequencing Meta Learning (TSML) model. The method can intelligently perform fault diagnosis on an industrial system through a meta-learning strategy.
[0038] Meta-learning is a highly adaptive learning strategy that does not focus on learning itself, but on how to acquire learning ability. With the help of the learning ability, it only needs simple adjustments to adapt to new tasks in real industrial scenarios, instead of training the network from scratch. Specifically, meta-learning does not d...
PUM
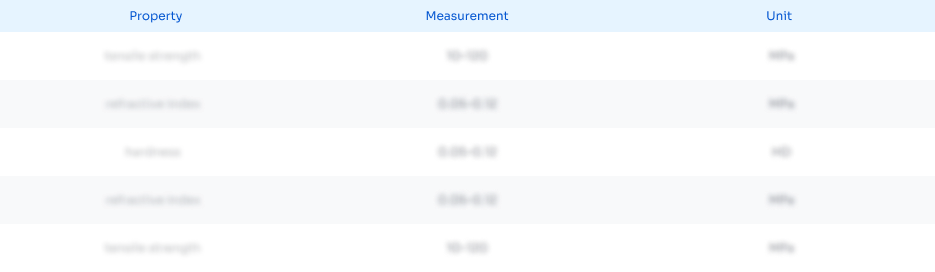
Abstract
Description
Claims
Application Information
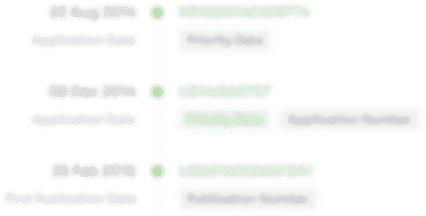
- R&D
- Intellectual Property
- Life Sciences
- Materials
- Tech Scout
- Unparalleled Data Quality
- Higher Quality Content
- 60% Fewer Hallucinations
Browse by: Latest US Patents, China's latest patents, Technical Efficacy Thesaurus, Application Domain, Technology Topic, Popular Technical Reports.
© 2025 PatSnap. All rights reserved.Legal|Privacy policy|Modern Slavery Act Transparency Statement|Sitemap|About US| Contact US: help@patsnap.com