Welding defect real-time detection method and system based on high-frequency time sequence data
A technology of time series data and welding defects, which is applied in image data processing, neural learning methods, image enhancement, etc., can solve the problems of non-parallel calculation, slow training speed, and long sequence length of cyclic neural network, and achieve strong practical significance and speed up Training speed, real-time better effect
- Summary
- Abstract
- Description
- Claims
- Application Information
AI Technical Summary
Problems solved by technology
Method used
Image
Examples
Embodiment
[0030] Such as figure 1 Shown is a real-time detection method for welding defects based on high-frequency time series data, including the following steps:
[0031] Step 1: Data preprocessing;
[0032] Step 1.1: Collect the high-frequency welding data (not lower than 10KHz, such as figure 2 As shown, the welding timing data in this embodiment includes current, voltage and airflow velocity), set the window length window_size=20000, each window length sequence is taken as a sample (a total of 1600 samples), and each sample is saved For a NumPy file, named data_i.npy;
[0033] Step 1.2: Label each sample according to the known defect occurrence time period and defect type, and save each label (label) as a NumPy file, named label_j.npy, i and j correspond one-to-one; where label There are three types:
[0034] label category 0 normal 1 Missing solder 2 Stomata
[0035] Step 1.3: Randomly shuffle all generated samples (out of order), set the p...
PUM
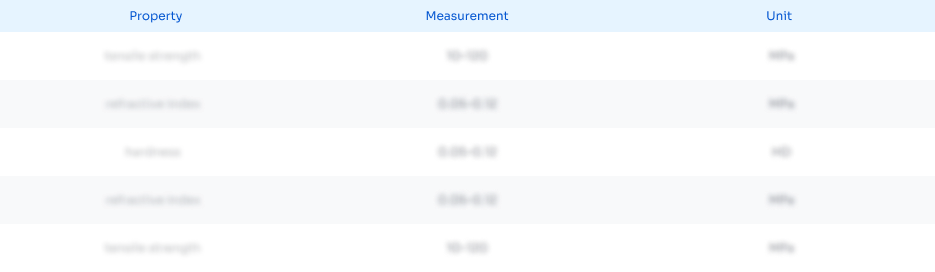
Abstract
Description
Claims
Application Information
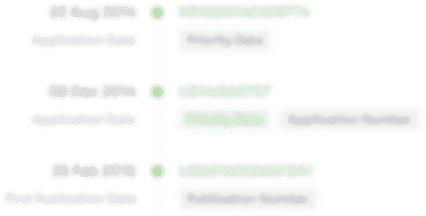
- R&D
- Intellectual Property
- Life Sciences
- Materials
- Tech Scout
- Unparalleled Data Quality
- Higher Quality Content
- 60% Fewer Hallucinations
Browse by: Latest US Patents, China's latest patents, Technical Efficacy Thesaurus, Application Domain, Technology Topic, Popular Technical Reports.
© 2025 PatSnap. All rights reserved.Legal|Privacy policy|Modern Slavery Act Transparency Statement|Sitemap|About US| Contact US: help@patsnap.com