Asynchronous federated learning method and device for improving utilization efficiency of edge device, and medium
An edge device and learning method technology, applied in machine learning, multi-program device, program control design, etc., can solve problems such as complex network environment, lower device utilization, loss of connection between edge devices and servers, and alleviate data competition Effects of relationship, utilization efficiency improvement, and concurrency performance improvement
- Summary
- Abstract
- Description
- Claims
- Application Information
AI Technical Summary
Problems solved by technology
Method used
Image
Examples
specific Embodiment 2
[0141] On the edge device side, there is still the problem of low utilization of edge devices. First, edge devices must meet some stringent conditions to start model training, for example: mobile phones should be in charging, standby, and can access the Internet through a wireless network etc., the purpose is to prevent the training process from disturbing the normal use of the mobile phone by the mobile phone user; secondly, the network environment where the edge device is located is complex, and the connection between the edge device and the server may be lost from time to time. The above two situations will cause the model training process to be terminated, causing the device to go offline and enter an idle state, further reducing the utilization rate of the device, resulting in the inability to fully utilize the concurrent performance of the server.
[0142] For this reason, the present invention provides the following specific implementation cases to solve the above proble...
PUM
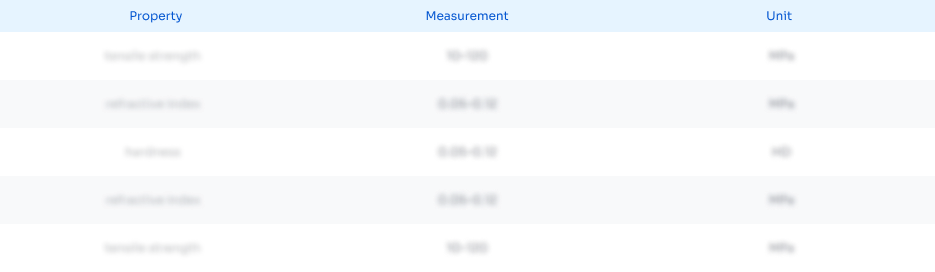
Abstract
Description
Claims
Application Information
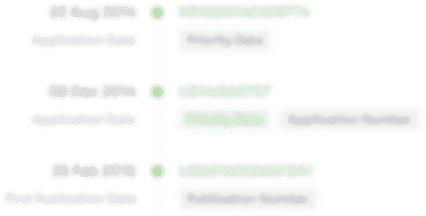
- R&D
- Intellectual Property
- Life Sciences
- Materials
- Tech Scout
- Unparalleled Data Quality
- Higher Quality Content
- 60% Fewer Hallucinations
Browse by: Latest US Patents, China's latest patents, Technical Efficacy Thesaurus, Application Domain, Technology Topic, Popular Technical Reports.
© 2025 PatSnap. All rights reserved.Legal|Privacy policy|Modern Slavery Act Transparency Statement|Sitemap|About US| Contact US: help@patsnap.com