Classification method based on hyper-graph transformation network
A classification method and neural network technology, applied in the field of deep neural network algorithm and node division, and graph mining, can solve problems such as inability to accurately classify, inability to deeply explore high-level node information in heterogeneous networks, etc., and achieve the effect of great application potential
- Summary
- Abstract
- Description
- Claims
- Application Information
AI Technical Summary
Problems solved by technology
Method used
Image
Examples
Embodiment Construction
[0065] The experimental environment of this method is: Ubuntu18.04.5, GeForce RTX 3090 24GB, CUDA=11.2, python=3.8.3, pytorch=1.7.1. The training set and test set are divided, and the division ratio is 0.8. The main parameters are set as: Epoch=500, learn rate=0.0005, weight decay=0.001.
[0066] Obtain the data of the paper, and make the data set into two parts: node feature X and graph structure. The node feature is the ONE-HOT code of the thesis title, and the graph structure describes the topology of the node. Incidence Matrix Available Matrix Indicates that the vertical axis of H represents the node v, and the horizontal axis represents the hyperedge e, where N is the maximum number of nodes, and M is the maximum number of hyperedges. The element H(v,e) in the matrix is defined as:
[0067]
[0068] calculate with Element D in the degree matrix of hypergraph node i v (i,i) is defined as:
[0069]
[0070] degree matrix D of hyperedge i e The inner elem...
PUM
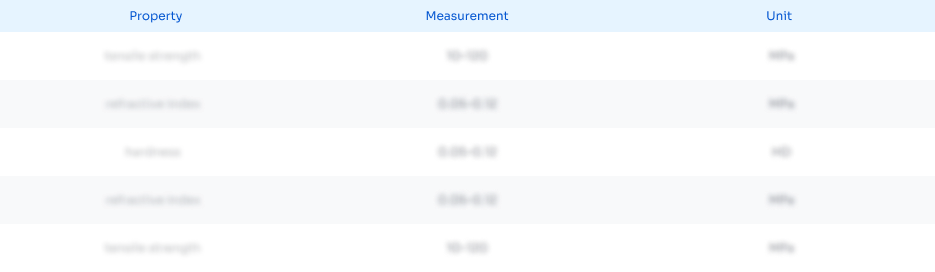
Abstract
Description
Claims
Application Information
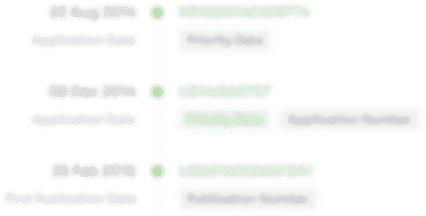
- R&D Engineer
- R&D Manager
- IP Professional
- Industry Leading Data Capabilities
- Powerful AI technology
- Patent DNA Extraction
Browse by: Latest US Patents, China's latest patents, Technical Efficacy Thesaurus, Application Domain, Technology Topic, Popular Technical Reports.
© 2024 PatSnap. All rights reserved.Legal|Privacy policy|Modern Slavery Act Transparency Statement|Sitemap|About US| Contact US: help@patsnap.com