Anterior chamber angle image grading method fused with weak supervision metric learning
A technology of metric learning and grading method, applied in the field of medical image processing, can solve the problems of low accuracy and low efficiency, and achieve the effect of strengthening learning, reducing interference, and efficient diagnosis and treatment plan
- Summary
- Abstract
- Description
- Claims
- Application Information
AI Technical Summary
Problems solved by technology
Method used
Image
Examples
Embodiment 1
[0057] Such as figure 1 As shown, a method for grading anterior chamber angle images that incorporates weakly supervised metric learning includes the following steps:
[0058] S1: Obtain anterior chamber angle images and perform image screening;
[0059] It should be noted that, at first, the images of the anterior chamber angle are collected, for example, at least 1000 images of the anterior chamber angle can be collected (such as figure 2 shown as the anterior chamber angle image), the collected images of the anterior chamber angle are screened, including: eliminating the images that cannot distinguish the grade of the anterior chamber angle due to objective conditions, and the images that cannot distinguish the grade of the anterior chamber angle due to objective conditions include : No images of anterior chamber angle structure, images with too strong light or too dark light with unclear structure of anterior chamber angle; images whose grade features are not obvious and...
Embodiment 2
[0076] In this embodiment, step S5 is described in detail based on the above steps.
[0077] S5: Use the training set and verification set to train and verify the constructed model to obtain the optimal network model;
[0078] Such as Figure 4 As shown, the specific process is:
[0079] S501: Select images for training from the training set, and perform data enhancement processing on the selected images;
[0080] The process of data enhancement is: perform random horizontal mirroring on the selected anterior chamber angle image with a probability of 0.5 (for example, randomly select 50% of the images to be enhanced for horizontal mirroring), and add salt and pepper noise to the image with a probability of 0.5 , where the signal-to-noise ratio is 0.95; the image is scaled; finally, the image is normalized and regularized, so that the image before the input model is in tensor format, and the value distribution is between [-1,1] ;
[0081] S502: Input the enhanced image data...
Embodiment 3
[0104] In this embodiment, the existing technology is divided into a traditional network and a weakly supervised network. The traditional network includes: VGG, GoogLeNet and ResNet. The traditional network consists of a large number of convolutional layers, pooling layers, batch normalization layers, and activation layers. Several classic models of convolutional neural networks; weakly supervised network methods are mainly divided into three types: consistency regularization, pseudo-label labeling, combined use of consistency regularization and pseudo-label labeling, these three methods are respectively provided by CCT, UPS , FixMatch as a representative method for comparative experiments, wherein Ours represents the method of the present invention, and the experimental results are shown in Table 1 and Table 2.
[0105] Table 1 Comparison table of classification performance on anterior chamber angle images and fundus images
[0106]
PUM
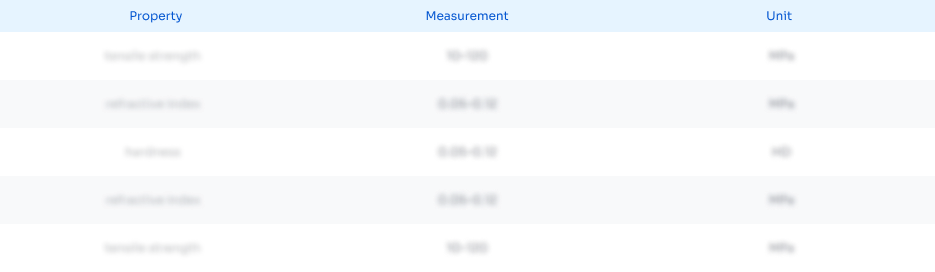
Abstract
Description
Claims
Application Information
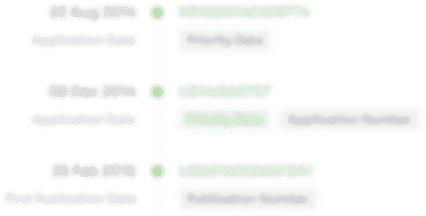
- R&D Engineer
- R&D Manager
- IP Professional
- Industry Leading Data Capabilities
- Powerful AI technology
- Patent DNA Extraction
Browse by: Latest US Patents, China's latest patents, Technical Efficacy Thesaurus, Application Domain, Technology Topic, Popular Technical Reports.
© 2024 PatSnap. All rights reserved.Legal|Privacy policy|Modern Slavery Act Transparency Statement|Sitemap|About US| Contact US: help@patsnap.com