Deep learning-based pulmonary tuberculosis recognition and diagnosis model, method and device and medium
A diagnostic model and deep learning technology, applied in the field of deep learning, can solve the problems of missing key details and wrong diagnosis, and achieve the effect of improving efficiency, application scope, and accuracy.
- Summary
- Abstract
- Description
- Claims
- Application Information
AI Technical Summary
Problems solved by technology
Method used
Image
Examples
Embodiment 1
[0059] The tuberculosis identification and diagnosis model based on deep learning of the present invention includes three strategy models, namely Concatenation strategy model, Voting strategy model and Attention strategy model.
[0060] The Concatenation strategy model is used to extract features from the input image to obtain a feature map, and perform global average pooling on the feature map to obtain a feature vector, which is used to perform dimension reduction on the obtained feature vector to unify the dimensions of the feature vector and unify the dimensions. The spliced feature vector is spliced to obtain the spliced feature vector, and the spliced feature vector is used to identify and classify through the fully connected layer to obtain the diagnosis result.
[0061] The Voting strategy model is used to extract features from the input image to obtain a feature map, and perform global average pooling on the feature map to obtain a feature vector. For each dime...
Embodiment 2
[0072] The method for identifying and diagnosing pulmonary tuberculosis based on deep learning of the present invention comprises the following steps:
[0073] S100. Construct a pulmonary tuberculosis diagnostic model, the pulmonary tuberculosis diagnostic model is the deep learning-based pulmonary tuberculosis identification and diagnosis model disclosed in Example 1;
[0074] S200. Acquire tuberculosis X-ray images, each tuberculosis X-ray image corresponds to a diagnostic label;
[0075]S300. Construct a data set based on tuberculosis X-ray images and labels thereof, and divide the data set into a training set and a test set;
[0076] S400. Using the tuberculosis X-ray image in the training set as input and the diagnostic label as output, train the three strategy models in the tuberculosis identification and diagnosis model to obtain a trained tuberculosis identification and diagnosis model;
[0077] S500. Using the tuberculosis X-ray images in the test set as input, ident...
Embodiment 3
[0083] The device of the present invention includes: at least one memory and at least one processor; at least one memory for storing a machine-readable program; at least one processor for invoking the machine-readable program to execute the method disclosed in Embodiment 2.
PUM
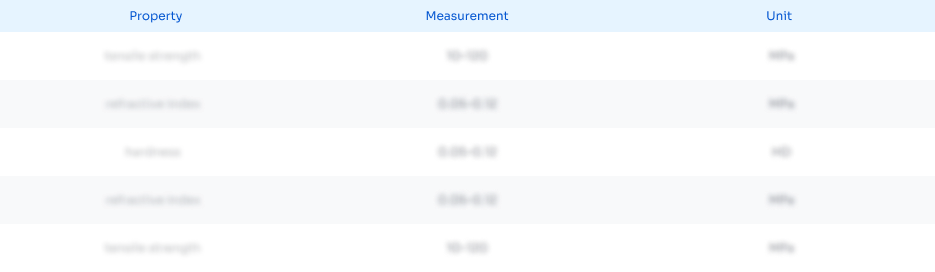
Abstract
Description
Claims
Application Information
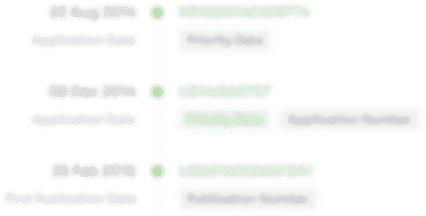
- R&D Engineer
- R&D Manager
- IP Professional
- Industry Leading Data Capabilities
- Powerful AI technology
- Patent DNA Extraction
Browse by: Latest US Patents, China's latest patents, Technical Efficacy Thesaurus, Application Domain, Technology Topic.
© 2024 PatSnap. All rights reserved.Legal|Privacy policy|Modern Slavery Act Transparency Statement|Sitemap